下载中心
优秀审稿专家
优秀论文
相关链接
摘要
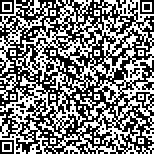
针对复杂PolSAR图像场景中机场目标区域检测问题,本文提出一种自适应无监督分类的机场目标快速检测方法,该方法首先对极化SAR相干矩阵分解提取PolSAR图像的特征值图,转化为超像素图实现图像去噪及降维。然后用SLIC超像素分割算法分割构造超像素。基于超像素图构建极化分类特征,并采用无监督的谱聚类方法提取出疑似机场跑道区域,其中的类别数确定利用VAT-DBE(Visual Assessment of cluster Tendency-Dark Block Extraction)算法获得。最后,在疑似区域内结合跑道结构特征进一步辨识检测出场景中的机场跑道区域。利用美国UAVSAR系统采集的多组全极化SAR实测数据对算法进行验证,并与两种已有的无监督跑道检测算法进行对比,实验结果表明,该算法能够快速准确检测出机场跑道区域,处理耗时可减小80%以上。具有更好的鲁棒性。
Airport detection is important for military and civilian applications. As a prominent feature of an airport, a runway is frequently used to recognize airport areas in image-processing fields. The classification method is extensively used to resolve the problem of airport detection in PolSAR images. That is, the runway area is extracted through the classification method to determine the airport area. A disadvantage in applying this method is that the number of class in the image must be manually set through the classification method. This process will decrease the robustness of the algorithm. Moreover, given that the classification is normally performed on the pixel of an image, a high computational cost is required. In this study, a fast, adaptive unsupervised classification method for runway detection is proposed.
First, the coherent matrix of a PolSAR image is decomposed to construct the eigenvalue image, and then the superpixel image is obtained when the eigenvalue image is processed through the SLIC algorithm. Second, the number of categories of ground objects in the superpixel image is estimated, and the runway ROIs of the superpixel image are extracted through the spectral clustering method, which is an adaptive unsupervised classification. Finally, the prospected areas are further filtered morphologically, and the real runways are identified by combining with the structural features, such as the size, topology, and parallel characteristics of the runways.
The algorithm is verified using multiple groups of real PolSAR images acquired through the UAVSAR system and compared with two representative unsupervised algorithms. Experimental results show that the proposed algorithm can detect the runway area quickly and accurately with low false alarm rate And that the runway structure is clear.
These experimental results are improved with the use of the proposed algorithm. Thus, the following conclusions are drawn:The SLIC algorithm is used to form a superpixel image to compress the dimension of the original image and reduce computational cost. Simultaneously, speckle noise is suppressed slightly, This result is beneficial for the classification in the subsequent processing. The adaptive class number estimation of images with VAT and DBE algorithms increase the robustness of the proposed method. Runways are extracted effectively and accurately when the polarimetric feature of a PolSAR image is combined with the spectral clustering method.
Further studies must be explored despite obtaining improved detection results through the proposed method. For example, improving the blurred part caused by a superpixel image in the detected airport area must be investigated. A probable scheme is to set the number of the superpixel of the image adaptively in accordance with the complexity of the original image contents to yield a high-quality superpixel image.