下载中心
优秀审稿专家
优秀论文
相关链接
摘要
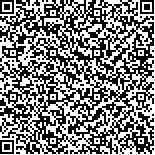
近年来,遥感应用的快速发展推动了遥感载荷指标性能的不断提升。但由于遥感传感器的硬件技术瓶颈,遥感数据无法同时具有高空间分辨率、高光谱分辨率、高时间分辨率的指标特性。遥感数据融合是解决该问题的有效方法。为了深入了解目前遥感数据融合技术的研究进展情况,本文对国内外1992年—2018年间在该领域有一定影响力的相关成果进行了调研、分析与归纳总结。首先对遥感数据融合相关论文的年发文量、发文国家与机构、发表刊物以及关键词等进行了统计,梳理其发展历史及趋势;系统性的总结了各类数据融合算法,将其分为面向空间维提升的融合算法、面向光谱维提升的融合算法以及面向时间维提升的融合算法3类,并对各类算法的优势与适用性进行了分析;归纳总结了遥感数据融合的质量评价指标,包括有参考影像的融合评价指标以及无参考影像的融合评价指标;最后对遥感数据融合进展进行了总结与展望。
Remote sensing applications have been promoting the development of satellite sensors and their performances. The satellite sensors are now possessing higher and higher data resolutions, and it in turn greatly facilitating remote sensing applications. The improvement of spatial resolution makes it possible to precisely record textures and spatial features of land covers. The improvement of spectral resolution makes it possible to precisely classify and retrieve parameters. The improvement of temporal resolution makes it easier to record the temporal changes of land covers during different phases. However, due to the limitation of sensors, satellite data can not possess high spatial resolution, high temporal resolution and high spectral resolution at the same time, which greatly hinders further remote sensing applications.
Remote sensing data fusion is one of the effective solutions to deal with the problem of limitation in sensors' resolutions, which is to integrate data from different sources and with different resolutions using algorithm methods to get richer information than one single image data. In recent years, the remote sensing data fusion methods have been greatly developed and related articles boost in growing numbers and importance. Thus, this article aims to systematically introduce the remote sensing data fusion and its current progress. Former reviews of remote sensing data fusion divides fusion methods into different categories. Based on processing levels, the remote sensing data fusion methods can be divided into pixel-level fusion, feature-level fusion and decision-level fusion. And based on the data sources, the remote sensing data fusion methods can be divided into homogeneous data fusion, heterogeneous data fusion, fusion for remote sensing observation and station data, and fusion for remote sensing observation and non-observed data. And this article adopts another category system which makes it more systematic and comprehensive in mathematical principles.
In order to systematically indicate the current progress and developed history of remote sensing data fusion methods, this article first did bibliometric analysis on the remote sensing data fusion articles from data sources from Web of Science (WOS) and Chinese National Knowledge Infrastructure (CNKI). The data was preprocessed and has removed duplicates. Analysis including the aspects of yearly publishments, countries and organizations, journals and key words to see the history and trends have been done by Histcite, Bibliometric, Citespace and Endnote. Then the remote sensing fusion methods are systematically introduced. According to different resolution improvements, this article firstly divided the fusion methods into three categories:fusion methods emphasizing improvements of spatial resolution development, spectral development and temporal development, and based on that we further divide them into subcategories according to different mathematical principles. And the basic principles, some of the important methods, the advantages and flaws of these methods are systematically introduced in this section. Also, we did detailed instructions on fusion result assessment methods including methods with reference image and without reference image. Under these two categories we further introduced different assessment metrics and its applicable scenarios. And finally, this article made summaries of these fusion methods and described its features and directions. And finally makes predictions of future trends in remote sensing data fusion methods.