下载中心
优秀审稿专家
优秀论文
相关链接
摘要
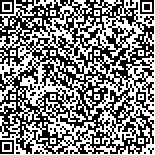
训练样本质量是决定农作物遥感识别精度的关键因素,虽然高空间分辨率卫星的发展有效地解决了农作物遥感识别过程中的混合像元问题,但是当区域内不同作物种植面积差异较大时,训练集中不同类别样本数量往往相差较大,这样的不均衡数据集影响分类器的训练,导致少数类别的识别精度不理想。为研究作物遥感识别过程中的不均衡样本问题,本文基于GF-2号卫星数据,首先挖掘了地物的光谱信息、纹理信息,用特征递归消除RFE (Recursive Feature Elimination)方法进行特征优选,然后从数据处理的角度采用了5种采样算法对不均衡训练集进行处理,最后使用采样后的均衡数据集训练分类器,对比数据采样前后决策树与Adaboost(Adaptive Boosting)两种分类器的识别结果,发现:(1)经过采样处理后两种分类算法明显提升了小宗作物的分类精度;(2)经过ADASYS (Adaptive synthetic sampling)采样处理后,分类器性能提升最多,决策树的Kappa系数提高了14.32%,Adaboost的Kappa系数提高了10.23%,达到最高值0.9336;(3)过采样的处理效果优于欠采样,过采样对分类器的性能提升更多。综上所述,选择合适的采样方法和分类方法是提高不均衡数据集遥感分类精度的有效途径。
The rapid development of high-spatial-resolution satellites has effectively alleviated the problem of mixed pixels in satellite images, thereby enabling extraction of the meticulous distribution of crops from them. The classification of remote sensing images is a quick way to obtain accurate agricultural information. However, the accuracy of supervised classification using remote sensing images is affected by several factors, such as classifier algorithm and input datasets. The imbalanced training samples, which indicates the number of training samples of some categories is considerably smaller or larger than the others, often results in poor classification accuracy for the minority classes. To improve this situation and generalization performance of classifier, this research focused on proper utilization of resampling techniques and classification methodologies for achieving perfect performance of remote sensing image classification.
We investigated the aforementioned images by data mining approaches including spectrum and texture features and selection of optimized features based on recursive feature elimination. Then, five resample methods, namely, three over-resampling methods and two under-sampling methods, were separately used to balance the initial training datasets. Finally, we tested the resampled datasets by utilizing two classifiers (decision tree and AdaBoost) and evaluated the performance of each one in terms of kappa coefficient, overall accuracy, producer's accuracy, and user's accuracy.
The overall classification accuracy and kappa coefficient improved considerably on decision tree (14.32%) and AdaBoost classifier (10.23%) after resampling. The AdaBoost obtained the highest value of kappa coefficient (0.9336) by using the training dataset resampled with ADASYN. The accuracy of classification on minority crops was also increased by resampling training datasets. Meanwhile, feature selection results showed that vegetation and texture indexes were more efficient than features of original reflection ratio to classification. Over-resampling methods had advantages in relieving the influence of imbalanced training samples to classifiers.
Resampling process to training datasets has remarkable advantage in improving the classifier performance if the training datasets are critically imbalanced. The detailed accuracy assessment shows that over-resampling method is more excellent than under-resampling. The reason is that some significant samples are lost during under-resampling, but helpful and useful information is added after over-resampling. AdaBoost classifier performs better than decision tree in terms of solving imbalanced training datasets. Combination of proper resampling approaches and compatible classifier can significantly improve the accuracy of minority classes in the situation of imbalanced dataset classification.