下载中心
优秀审稿专家
优秀论文
相关链接
摘要
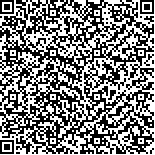
在遥感影像结合矢量数据先验信息的变化检测中,需要从分割后的影像对象中抽取一定数量、具有相同类别属性的样本,其中不可避免地抽到类别属性不一致的样本,如何剔除这些样本是抽样过程中必须解决的重点问题,在目前已有的方法中,一般是通过人工目视判别完成的。样本的自动提取是实现自动变化检测的关键环节,本文提出一种变化检测样本自动抽样方法,主要包括样本的空间布设和异常样本自动检测两个环节。该方法首先利用矢量数据提取抽样图层,用抽样图层分割遥感影像,获取影像对象。其次是根据抽样区域范围、影像对象分布特征和地形特征布设变化检测样本。然后根据样本的先验类别属性构建特征空间向量,计算样本在特征空间的局部可达密度,由局部可达密度计算样本的异常度指数,并根据特征空间密度异常指数剔除异常样本,完成变化检测样本自动提取。最后以耕地、林地和居民地为例进行了抽样试验。结果表明,邻域参数k按样本布设总数的1/5—1/3取值、异常度阈值设定为80%时,可以实现异常样本0漏检率,能够准确、高效实现变化检测样本的自动提取。
Some existing classification vector datasets are available for change detection in many regions, and some prior knowledge-such as position, shape, size, and class-are included in these datasets. The change detection method, which rely on remote sensing images and vector datasets, has become an important research focus. The automatic sampling method for the change detection of samples is the key technology in achieving automatic change detection. Therefore, an automatic sampling method based on the feature space density outlier index is proposed.
The change detection of vector data/remote sensing images is realized by focusing on the spectral feature difference between the image objects needed to be detected and the samples. On the one hand, the samples must be able to differentiate the regional environment characteristics in a spatial distribution, and this aspect belongs to the problem realm of the sampling distribution method. On the other hand, the samples must include the image objects with no changes in the posterior class attribute, and this aspect is important in the detection of outlier samples. The automatic sampling method proposed in this study includes the spatial layout of samples and the automatic detection of outlier samples. The reasonable spatial distribution of samples is the precondition for improving the accuracy of change detection results, and an automatic detection method for the outlier samples is an important part of automatic change detection technology.
In acquiring the samples for change detection, we initially extract a sampling layer from the vector data and use this layer to segment the remote sensing image. In this manner, the image objects can be extracted. Second, samples are extracted from the image objects, and we determine whether the spatial distribution of these samples directly yet reasonably affects the accuracy of the change detection results. On the one hand, the rationality of the sample space layout should reflect the correctness and uniqueness of the samples in typical normal areas. On the other hand, the sample layout must be able to reflect the overall features of the image objects. The sample layout must also be random and uniform, and the samples should be representative. Thus, we extract samples of the image objects in accordance with the sampling region and by considering the distribution and topographic features of these image objects.
Then, we detect the outlier image objects in the samples. Local reachability density (LRD) is applied to quantitatively describe the intensity of an image object relative to its neighborhood's image objects in the feature space. In particular, the LRD algorithm is used to construct an outlier index that can measure the outlier degree of the sample image objects in the feature space. Feature space vectors are also constructed on the basis of the texture feature parameters. Then, the LRDs of the samples are calculated according to reachability distance. The feature space outlier index (FSOI) is also defined and then used to detect the outlier sample objects.
Finally, an experimental research on the proposed sampling method was carried out by using a vector topographic map and a high-resolution remote sensing image. The experimental results indicate that the full detection of outlier samples can be achieved when the k value is set from 1/5 to 1/3 of the total number of samples and the FSOI threshold is set to 80%. The proposed sampling method can effectively achieve automatic sampling of change detection samples.