下载中心
优秀审稿专家
优秀论文
相关链接
摘要
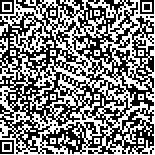
高光谱图像异常点检测中,传统RX异常点检测算法忽略了空间相关性,背景估计不准确。本文提出了一种基于图像局部邻域光谱显著性分析的加权RX算法。该算法通过引入图像显著性分析,对基于概率密度为权重的图像背景建模进行改进,建立光谱显著性权重图,重新定义RX算法中的均值向量和协方差矩阵,并给不同的目标赋予不同的权值,达到优化背景估计的目的。利用合成高光谱数据和真实高光谱数据进行异常点检测实验,结果表明,对于同一组数据,本文算法检测到的异常点数比传统算法多,虚警率较低,有效地提高了检测率。
With the development of spectral imaging technique and its data processing technology, anomaly detection using hyperspectral data has become a popular topic. Anomaly detection refers to the search for sparse pixels of unknown spectral signals in hyperspectral imagery. Given that the anomaly detection is unsupervised, providing a priori information is necessary. Thus, anomaly detection has a strong practicality. Considering the lack of spatial correlation and low normal distribution adaptation, the traditional RX algorithm has an inaccurate background estimation. Thus, this algorithm is unsuitable for detecting hyperspectral data.
In this study, a saliency weighted RX algorithm is proposed on the basis of the local neighborhood spectra of an image. When the human eye observes an image, the first object that is viewed is frequently the most significant. The significance of the saliency detection algorithm is to identify this goal. The saliency map is a 2D image of the same size as the original image to represent the significance of the corresponding pixel in the original image. In this algorithm, the image background modeling based on probability density is improved by introducing a saliency analysis method. Afterward, the spectral saliency map is established, and the mean vector and covariance matrix of the RX algorithm are redefined. Saliency weighted RX algorithm provides different weights to optimize the background estimation.
Anomaly detection experiments are conducted using synthetic and real hyperspectral data. Synthetic data experimental results show that, for each target, the number of anomalies detected using the saliency weighted RX algorithm is more than that of the traditional algorithms, and the saliency weighted RX algorithm can detect anomalies with abundance below 0.1. By contrast, traditional algorithms cannot detect these anomalies. Moreover, the false alarm pixels of the traditional algorithms are distributed in various positions, whereas the saliency weighted RX algorithm concentrates on an area called a false alarm area. This area can be removed effectively by morphological filtering. Real data experimental results show that the saliency weighted RX algorithm corresponds to the largest AUC value and has the optimal detection results.
The traditional RX algorithm assumes that the background model follows a multivariate Gaussian distribution and does not perform well in hyperspectral imagery. The method of saliency analysis in the field of computer vision can be effectively analyzed in the spatial domain. This phenomenon compensates for the shortcomings of the RX algorithm to ignore spatial correlation, thus detecting the anomalies synchronized in the spatial and spectral domains. The saliency weighted RX algorithm uses a saliency analysis method to provide the background and anomaly pixels with a different weight, thereby improving the adaptability of the background model. Through the experiment of synthetic and real data, the saliency weighted algorithm can improve the detection probability while reducing the false alarm rate in comparison with the traditional RX algorithm and has a certain anti-noise ability.