下载中心
优秀审稿专家
优秀论文
相关链接
摘要
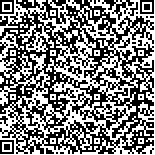
高光谱图像类内光谱变化较大,“同物异谱”现象普遍存在。利用原始地物光谱特征进行分类精度较低而且分类结果图中存在“椒盐现象”。为了获得好的分类结果,必须充分利用高光谱图像的光谱信息和空间信息,减少类内的光谱变化,并扩大类别间的光谱差异。为此,提出一种滚动引导递归滤波的高光谱图像光谱—空间分类方法。首先,利用主成分分析对高光谱图像进行降维;然后,利用高斯滤波对输入图像进行模糊化,消除图像中的噪声和小尺度结构;接下来,将模糊化后的图像作为引导图像,对输入图像进行边缘保持递归滤波,输出结果作为新的引导图像,重复迭代这个过程直至大尺度边缘被恢复;最后,利用提取的特征波段和支持向量机对高光谱图像进行分类。在两个真实高光谱数据集上进行了分类实验,结果表明本文方法的分类精度优于其他的高光谱图像分类方法。在训练样本极少的情况下,本文方法也能获得较高的分类精度。
Hyperspectral images have relatively large changes in intra-class spectra, and the "same material, different spectra" phenomena are widespread. Moreover, classification accuracy is low when only the original spectral features are considered, and the "pepper and salt phenomenon" can be observed in the classification result map. In the attempt to improve the classification result, the spectral and spatial information of a hyperspectral image needs to be fully utilized to reduce the spectral changes within the class and expand the spectral difference between classes. Thus, in this study, we propose a spectral-spatial hyperspectral image classification method with rolling guidance recursive filtering.
First, principal component analysis (PCA) is used to reduce the dimension of the hyperspectral image, in which the main components with large amounts of information are maintained for the input image of the next step. Second, the Gaussian filter is adopted to blur the input image and eliminate the small-scale structure. Third, recursive filtering is performed on the input image to preserve the edge of the image, in which the guidance image is the blurred image, and then the output result is treated as the new guidance image. The process is repeated iteratively until the large-scale edge is restored. Finally, the extracted feature bands of the hyperspectral image are used for classification by a support vector machine.
Indian Pines and University of Pavia datasets were initially used to verify the effectiveness of the proposed rolling guidance recursive filtering (RGRF) method. As shown by the homogeneous regions in Figs. 5 and 6, the noise phenomenon is severe when the extracting feature of PCA is used, whereas the noise is effectively removed when rolling guidance filtering (RGF) and RGRF are utilized. However, at the strong edges, the boundary is blurred and irregular and the spatial smoothness is excessive for RGF. By contrast, the boundary is clear and the strong edges are protected for RGRF. Then, the proposed method is compared with related classification methods by using two real hyperspectral image datasets. The comparative results in Tables 1 and 2 indicate that RGRF can improve classification accuracy more effectively than the other methods. Subsequently, McMemar test was performed to analyze the statistical significance and verify the comparative results. As shown in Tables 3 and 4, the correlations of RGRF with the other methods are statistically significant, and the values exceed p>1.96. The McMemar values in Tables 3 and 4 increase with the decrease in number of samples, which indicates that the advantages of using RGRF becomes more obvious in the case of fewer samples.
This study presents an improved edge-preserving filtering method named RGRF. Compared with the other filtering methods, RGRF can maintain the edge structures of land covers and eliminate the texture and noise information within these land covers. RGRF effectively uses the recursive filtering method instead of the bilateral filtering method (i.e., of the RGF method) in each iteration. In this manner, the continuous spreading of texture and noise information across the strong edges is avoided. Moreover, considering that image quality is significantly improved after RGRF filtering, the classification accuracy of the hyperspectral image is also greatly enhanced. Classification experiments were performed on two real hyperspectral datasets. The results indicate that the classification accuracy of RGRF is superior to the other hyperspectral image classification methods. Moreover, RGRF can achieve higher classification accuracy when only few training samples are used.