下载中心
优秀审稿专家
优秀论文
相关链接
摘要
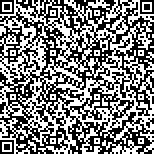
以北方典型河口湿地—黄河三角洲湿地为研究区,采用在特征选择和分类提取等方面具有明显优势的随机森林算法,对研究区内的湿地信息进行提取。首先基于多时相、光谱信息丰富的Sentinel-2数据生成4类不同的特征变量,包括光谱特征、植被指数和水体指数、红边指数、纹理特征;再根据以上特征构建6种不同的提取方案,对黄河三角洲湿地信息进行提取并验证不同方案的提取精度,旨在选择最佳方案改善湿地信息提取的效果。结果表明:(1)有效地使用多种特征变量是提高湿地信息提取的关键,就不同特征对湿地信息提取的贡献率而言,红边指数 > 植被指数和水体指数 > 光谱特征 > 纹理特征;(2)基于随机森林算法优选的特征变量提取效果最佳,总体精度高达90.93%,Kappa系数为0.90,表明随机森林算法可以有效地进行特征选择,在特征变量数据挖掘的同时,仍能保证湿地信息提取的精度,提高运行效率。本研究为湿地信息提取在数据源选择、特征选择和方法选择方面提供了一种新思路、方法和技术手段。
Estuary wetland is a special wetland type, and the extraction of estuary wetland information plays an important role in wetland conservation and scientific research. In this study, Yellow River Delta wetlands, as a typical estuary wetland in the north part of China, are considered the study area. The random forest method, which has evident advantages in feature selection and classification, was chosen to extract wetland information from the study area. First, five different characteristic variables, namely, spectral features, vegetation index, water index, red edge index, and texture features, were generated based on Sentinel-2 data with rich multi-temporal and spectral information. Then, six different classification schemes were constructed based on the preceding characteristic information. Finally, random forest classifier was used to extract the wetland information of the Yellow River Delta and verify the extraction accuracy of different results. The purpose is to select the best plan to improve the effect of wetland information extraction. Results are as follows: (1) The effective use of multiple feature variables is the key to improving the extraction of wetland information. The contribution of different characteristics to the wetland information extraction is described as follows: the red edge index > vegetation index and water index > spectral feature > texture feature. (2) The preferred features based on the random forest algorithm are crucial to extraction accuracy, with an overall accuracy of up to 90.93%, and Kappa coefficient of 0.90. This result shows that the random forest algorithm can effectively process feature selection. In feature variable data mining, the accuracy of the wetland information extraction can be guaranteed, and the operation efficiency can be improved. This study also provides a new idea, method, and technology for the selection of data sources and feature and method selections for wetland information extraction.