下载中心
优秀审稿专家
优秀论文
相关链接
摘要
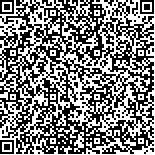
竹林是中国亚热带地区特殊而重要的森林资源,现有方法难以实现全国范围竹林时空分布信息快速准确提取。针对此问题,本研究利用2003年、2008年、2014年MODIS NDVI、反射率产品数据和省域Landsat分类数据,提出了基于决策树结合混合像元分解的全国竹林信息提取方法。首先,通过最大似然法获取中国林地分布信息;然后,在林地信息的基础上,构建决策树模型提取中国竹林分布信息;最后,采用线性最小二乘法混合像元分解得到中国竹林丰度图,并计算竹林面积。研究结果表明:(1)最大似然法提取的3个时期中国林地信息的生产者与用户精度均在90%以上,Kappa系数均值为0.93,为竹林信息提取奠定了基础。(2)C5.0算法构建的决策树模型能够很好的提取中国竹林时空分布信息,3个时期竹林分类精度均在80%左右。(3)在混合像元分解的基础上,统计得到的全国各省竹林估算面积与清查面积具有较高的相关性,R2分别为0.98、0.97和0.95,RMSE范围为3.92万—9.58万ha,说明估算得到全国竹林面积与实际情况较为吻合。本研究所提出基于MODIS遥感数据运用C5.0算法决策树结合混合像元分解的方法,实现了全国竹林时空分布信息的准确提取,为全国竹林资源信息动态监测及管理提供了技术手段和数据支撑。
A bamboo forest is a special and important forest resource that is distributed in subtropics of China. However, present methods cannot quickly and accurately extract spatiotemporal distribution information of a bamboo forest at the national scale.
This study presents a method for extracting bamboo forest information in China by combining decision tree and linear spectral unmixing methods using MODIS NDVI, reflectance product, and provincial Landsat classification data in 2003, 2008, and 2014. The steps are as follows:First, forest information was extracted based on the maximum likelihood method; second, a decision tree model was constructed to extract bamboo forest distribution information based on a forest information map; finally, the linear spectral unmixing method was applied to obtain the abundance map of a bamboo forest in China, and the bamboo forest area was calculated.
The main experimental results are as follows:(1) The forest information in China was extracted through the maximum likelihood method. The accuracy of a producer and a user was higher than 90%, and the Kappa coefficient was 0.93, thereby establishing the extraction of bamboo forest information. (2) The decision tree constructed by using a C5.0 algorithm satisfactorily extracted the bamboo forest spatiotemporal distribution information in China, with an average classification accuracy of 80%. (3) The estimated bamboo forest area of each province in China was highly correlated with the observations, with the R2 values of 0.98, 0.97, and 0.95, correspondingly. The RMSE ranged from 3.92×104 to 9.58×104 ha, thus indicating that the estimated bamboo forest area was essentially consistent with the actual situation.
In this study, the C5.0 algorithm decision tree, which is based on the MODIS remote sensing data, combined with the mixed pixel decomposition presents accurate extraction of bamboo forest spatiotemporal distribution information in China. This method provides the technical approach and data support for the dynamic monitoring and management of bamboo forest resources.