下载中心
优秀审稿专家
优秀论文
相关链接
摘要
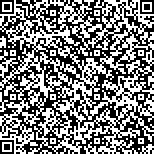
2. 北京师范大学 地理科学学部, 北京 100875
东北黑土区是中国重要的粮食生产区,而长期的开垦造成了严重的水土流失现象,坡耕地表面出现大量的侵蚀沟。侵蚀沟的识别是土壤侵蚀监测的重要手段之一,目前遥感技术在侵蚀沟的识别中应用广泛,但自动化程度不高。针对特定地物影像的识别,如何选取最能够有效描述该地物的特征是解决问题的关键。本文构建了耕地和侵蚀沟遥感影像的训练样本集,基于样本集分别提取了由光谱特征和纹理特征组成的浅层特征、SIFT特征经编码后得到的中层特征,以及利用卷积神经网络提取的深层特征;再基于不同层次的特征选用合适的分类器对遥感影像进行分类,识别出含有侵蚀沟的遥感影像,形成了一套针对侵蚀沟的特征提取与识别方法,为东北黑土区的耕地保护提供有力支持。测试结果表明:基于中层特征的识别精度最高,为98.5%,但该特征需要人工设计,自动化程度有限;而利用卷积神经网络可自动提取深层特征,其识别精度达到了95.5%,同时大大提高了自动化程度,满足侵蚀沟影像的识别的需求。
Northeast China is a major commodity grain base in China. The protection of cultivated lands in Northeast China is crucial for safeguarding the food security in China. The recognition of erosion gullies is an important means of monitoring soil erosion. Furthermore, remote sensing technology is extensively used in this field given the multiple advantages of this technology. However, the traditional methods based on remote sensing mostly depend on manual interpretations. Therefore, the degree of the automation and the efficiency are relatively low. In this study, multi-level features were extracted, thereby effectively describing the specific objects by using machine and deep learnings, and erosion gullies were identified based on these features to improve the accuracy and efficiency of recognizing erosion gullies.
In this study, we first cut the remote sensing images in a fixed size and labeled these images manually to create datasets as training samples that consist of two categories, namely, farmland and erosion gully. Second, we extracted spectral and textural features based on this dataset as low-level features, encoded SIFT features through ScSPM as middle-level features, and extracted high-level features by using CNN. Third, a linear SVM and a softmax classifier were applied to classify the remote sensing images based on the multi-level features to identify the images with erosion gullies. Finally, we completed a set of methods to extract the feature and recognize the erosion gully, thereby providing a robust support for protecting arable land in the black soil area of Northeast China.
The multi-level features extracted through the proposed method demonstrate specific capabilities in identifying erosion gully images. In the test phase, results show that the recognition outcome based on low-level features exhibits the lowest accuracy (91.1%), whereas the recognition accuracy based on middle-level features is the highest (98.5%). However, both features require a manual design. Hence, the degree of automation is limited. By contrast, the CNN can extract high-level features and automatically achieve an "end-to-end" learning, which highly improves the degree of automation of erosion gully recognition. Furthermore, the recognition accuracy based on high-level features is 95.5%, which satisfies the expectation of this study.
The recognition accuracy is slightly lower in the validation phase than in the test phase because the images typically contain several irrelevant objects in the practical application, thereby preventing the improvement of the accuracy. However, the proposed method can generally identify the erosion gullies in the images with a reasonable practicality.
Low-level features demonstrate several advantages, such as simple calculation and low time consumed. However, the capability to describe the erosion gully is relatively poor, thus resulting in low recognition accuracy. By contrast, the methods based on middle- and high-level features can identify nearly all the erosion gullies in the images, although these methods are time-consuming during the early training phase. Specifically, the method based on high-level features can automatically recognize the erosion gully. This study shows that deep learning has a great potential in remote sensing image application. If the sample size is continuously increased and the network structure expanded, then the recognition accuracy of erosion gully can be further improved.