下载中心
优秀审稿专家
优秀论文
相关链接
摘要
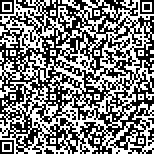
热带气旋TC(Tropical Cyclone)是全球影响最严重的自然灾害之一。TC强度和路径的准确预报,对于减轻其带来的灾害影响至关重要。本文基于静止红外卫星云图和相关向量机RVM(Relevance Vector Machine)构建有眼TC客观定强模型。首先,利用高斯平滑对红外卫星云图进行去噪;然后,利用基于测地活动轮廓GAC(Geodesic Active Contour)模型的偏微分方程PDE(Partial Differential Equation)法对有眼TC的眼壁进行分割,提取眼壁的亮温梯度信息,计算眼壁亮温梯度的最大值及梯度数据不同概率时的均值,从而构造与TC强度密切相关的特征因子;最后,利用RVM构建单特征因子、多特征因子与近地面最大中心风速的客观定强模型,研究不同特征维度对TC客观定强误差的影响。实验结果表明,在单特征因子的模型定强中,95%概率眼壁亮温梯度均值的定强误差最小,相比利用单特征因子所构建的定强模型,多特征因子的模型定强误差更小,即多特征因子中包含更多与TC强度相关的特征信息。在多特征因子的模型定强中,二特征因子优于三特征因子模型,说明应当合理选择特征因子维数,并非越多越好。本文所用RVM模型具有良好的高维非线性处理能力,能对TC强度进行有效估计。
A Tropical Cyclone (TC) is an influential natural hazard in the world. Thus, the forecasting accuracy of intensity and track is important to reduce the impacts of this disaster. In recent years, a remote sensing satellite cloud image gradually becomes the main means of monitoring a TC. In this study, an eyed TC intensity objective estimation model based on geostationary infrared satellite cloud image and Relevance Vector Machine (RVM) is proposed. First, the infrared satellite cloud image is denoised through a Gaussian smoothing method. Second, a TC eye area is segmented using a Partial Differential Equation (PDE) based on a Geodesic Active Contour (GAC) model. Third, the brightness temperature gradient data of the eyewall are obtained, and the maximum of brightness and different mean brightness temperature gradients with various probabilities are calculated. Thus, the characterization factors that are closely related to the TC intensity are structured. Finally, the TC intensity objective estimation model based on the RVM is established (single and multiple characterization factors) to study the influence of different characteristic dimensions on a TC intensity estimation error. Experimental results show that the estimation model that is established using the mean brightness temperature gradient of the TC eyewall with a probability of 95% performs better than the other models in studying intensity estimation model that is established using a single characterization factor. The error of the intensity estimation model established using multiple characterization factors is lower than that of the single characterization factor. That is, the multiple characterization factors contain additional characteristic information that is related to the TC intensity. In the study of the intensity estimation model established using multiple characterization factors, the estimation model performs better in the two characterization factors (the maximum of brightness and mean brightness temperature gradients with a probability of 95%) than in the three characterization factors. Therefore, not all "more" is better. Hence, the dimension of the characterization factor should be reasonably selected. Three types of RVM kernel functions are used in the experiments. Results show that all RVM kernel functions are efficient in estimating the TC intensity. Cauchy kernel function is suitable for the estimation model established using multiple characterization factors. The RVM model proposed in this study has a favorable high-dimensional nonlinear processing capability. Therefore, this model can effectively estimate the TC intensity.