下载中心
优秀审稿专家
优秀论文
相关链接
摘要
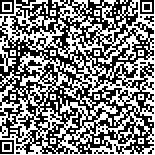
非负矩阵分解(NMF)技术是高光谱像元解混领域的研究热点。为了充分利用高光谱图像中丰富的空间与光谱相关性特征,改善基于NMF的高光谱解混算法性能,提出一种结合了空间与谱间相关性分析的NMF解混算法。算法针对NMF的通用性和局部极小问题,引入并结合高光谱图像两种典型的相关性特征,具体包括:基于马尔可夫随机场(MRF)模型,建立描述相邻像元空间相关特征的约束;通过复杂度映射技术,建立描述相邻波段谱间相关(光谱分段平滑)特征的约束;并将上述两种约束同时引入NMF解混目标函数中。实验结果表明,对于一般自然地物场景或人造地物场景,相对于分段平滑和稀疏约束的非负矩阵分解(PSNMFSC)、交互投影子梯度的非负矩阵分解(APSNMF)和最小体积约束的非负矩阵分解(MVCNMF)这3种代表性NMF解混参考算法,该算法可进一步提高高光谱解混精度;对于空间相关或谱间相关特征中某一种不显著的特殊场景,也具有更好的适应能力。通过将空间相关和谱间相关特征相结合,较全面地反映了高光谱数据与解混相关的重要特征,能够对绝大多数真实高光谱数据进行高精度解混,对高光谱解混及后续应用领域相关研究均具有参考价值。
The low spatial resolution of hyperspectral images always leads to the "mixed pixel" problem, where multiple objects exist in one pixel. The unmixing of mixed pixels is vital to the quantitative application of hyperspectral remote sensing. Non-negative matrix factorization (NMF) is a hot spot in hyperspectral unmixing research because of its non-negative constraints and capability to process high-dimensional data. However, NMF-based unmixing algorithms are ineffective because of universality, local minima, and other restrictions; additional specific features of hyperspectral images should be explored. Hyperspectral remote sensing images have significant correlation features, such as spatial and spectral correlation. The present study aims to improve the unmixing effect by adopting correlation features of hyperspectral remote sensing images.
An NMF-based hyperspectral unmixing algorithm with spatial and spectral correlation analyses, named NMF hyperspectral unmixing algorithm based on spatial and spectral correlation (NMFSSC), is proposed to address the universality and local minima problems of NMF. The proposed method adopts the correlation features of hyperspectral data in the unmixing process as the spatial correlation constraint of building adjacent pixels. The Markov Random Field (MRF) model and spectral correlation (piecewise smoothness) are used as constraints in building adjacent bands with complexity mapping technique. In this algorithm, the spatial correlation constraint of adjacent pixels is processed as the parallel and alternate step of the standard NMF object function. The spectral correlation of the adjacent bands is built as a new inner constraint of the standard function. During each iteration of the unmixing procedure, the distribution error of endmembers can be revised by the MRF-based spatial correlation constraint. The local minima problem can be revised by the complexity mapping constraint, thus potentially improving overall unmixing precision.
Actual hyperspectral data with high and low spatial correlation are adopted in the experiments. Three algorithms, namely, Minimum Volume Constrained Nonnegative Matrix Factorization (MVCNMF), Piecewise Smoothness NMF with Sparseness Constraints (PSNMFSC), and NMF with Alternating Projected Subgradients (APSNMF), are used with NMFSSC in the experiments. Results indicate that the proposed NMFSSC algorithm can improve unmixing precision, especially when dealing with high-spatial-correlation test data. The unmixing precision of NMFSSC is lower on the low-spatial-correlation data than on the high-spatial-correlation set, but the method still shows certain advantages over the three reference algorithms. Therefore, NMFSSC has a wide-ranging application scope.
In summary, the proposed algorithm can increase the unmixing accuracy of most actual hyperspectral remote sensing data, especially those with high spatial correlation. The local minima and universality problems of the standard NMF can be significantly addressed by adopting the MRF model and complexity mapping technique. However, the precision of the proposed algorithm can deteriorate as the correlation feature of hyperspectral data becomes increasingly vague. If the spatial and spectral correlations of the data are low, then the precision of the algorithm can be reduced. The next step is to widen the application scope of the unmixing algorithm and stabilize its performance.