下载中心
优秀审稿专家
优秀论文
相关链接
摘要
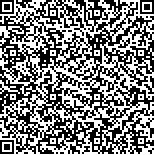
卫星气溶胶光学厚度(AOD)反演中,传统暗目标方法在反射率较低的水体、浓密植被覆盖区域取得了较好效果,在反射率较高且结构复杂的高反射地表上空目前多采用深蓝算法,但存在空间分辨率较低,对细节分布描述性较差等问题。为解决这一问题,本文首先以5年(2008年-2012年)长时间序列MODIS地表反射率产品为基础,采用最小值合成法建立500 m分辨率逐月地表反射率产品数据集,然后利用地物波谱库中典型地物波谱数据,分析建立MODIS与Landsat 8 OLI传感器蓝光波段反射率转换模型,最后北京地区AERONET地基观测数据确定了气溶胶光学物理参数,并反演获取了北京地区上空500 m分辨率的AOD分布。为验证反演算法的精度,分别将反演结果同AERONET及MODIS/Terra气溶胶产品(MOD04)进行交叉对比,同时利用相关系数R,均方根误差RMSE,平均绝对误差MAE以及MODIS AOD产品预期误差EE共4个指标进行衡量。结果表明:算法反演获取的AOD与AERONET观测值具有较高的一致性,各指标分别为R=0.963,RMSE=0.156,MAE=0.097,EE=85.3%,稍优于MOD04产品(R=0.962,RMSE=0.158,MAE=0.101,EE=75.8%),并且有效的对比点数也高于MOD04。通过与地基观测相比,卫星遥感获取的高分辨率城市地区AOD精度可作为定量评估城市空气质量的有效依据。
Satellite remote sensing has been widely used to retrieve Aerosol Optical Depth (AOD), which is an indicator of air quality and radiative forcing. The Dark Target (DT) algorithm is applied to low reflectance areas, such as vegetated or water areas, and the Deep Blue (DB) algorithm is adopted over bright-reflecting source regions. However, the spatial resolutions of the AOD products obtained with the DT and DB algorithms are relatively low, and the distribution details for urban areas are poor. In this study, a modified retrieval algorithm is proposed for the retrieval of AOD at a spatial resolution of 500 m over Beijing, China, on the basis of Landsat 8 OLI data. The key points are the accurate estimation of surface reflectance and the reasonable assumptions of the aerosol model.We developed a new algorithm to improve the accuracy of land surface reflectance for urban areas. A monthly Minimum Land Surface Reflectance (MLSR) database for China was established using Moderate Resolution Imaging Spectroradiometer (MODIS) surface reflectance products. The construction of this database was based on the principle of minimum synthesis technique. The conversion model from the blue band reflectance of MODIS to Landsat 8 OLI was established using the ASTER spectrum database to compensate for the difference between the spectral settings of the two sensors. AErosol RObotic NETwork (AERONET) data were adopted to determine aerosol optical properties, such as Ångström exponent, complex refractive index, Single Scattering Albedo (SSA), and asymmetric factor (g).The AODs retrieved from the 36 OLI images were validated with the AERONET data and the NASA/MODIS Collection 6 aerosol products at a 10 km resolution. For this purpose, the DT and DB (DT/DB) algorithms were combined. Results indicated that the proposed algorithm accurately retrieved AODs over the Beijing area and that the retrieved aerosol distribution contained more spatial details and variability than the DT/DB AOD products. Ground-based AERONET observations from four sites (Beijing, Xianghe, Beijing_CAMS, and Beijing_RADI) were used to validate the retrieved AODs. The results from the proposed algorithm demonstrated the highest accuracy, with an average correlation coefficient (R) and Root-Mean-Square Error (RMSE) of approximately 0.963 and 0.156, respectively. Approximately 85.3% of the points fell within the expected error (EE) ± (0.05+0.15 AOD) envelope defined by NASA. The DT/DB AODs showed a small R (0.962) and slightly large RMSE (0.158), with 75.8% of the collocations falling within the EE.An aerosol retrieval algorithm for Landsat 8 OLI 500 m data was proposed in this study. The assumptions in this algorithm are as follows: the variation of surface reflectance needs to be small for a month, and the SSA and g are regionally constant for a particular day. The method can also be used to achieve AOD inversion of other terrestrial observation satellite data. However, according to the uncertainty analysis, the proposed algorithm has some limitations that must be addressed. (1) Errors may arise from the use of constant SSA and g values for the day of retrieval. (2) The MLSR database approach was sometimes unsuccessful over snow surfaces, particularly when seasonal changes such as the snow melting in March and the accumulation of snow in Novembers are significant. These factors will be explored in our future studies.