下载中心
优秀审稿专家
优秀论文
相关链接
摘要
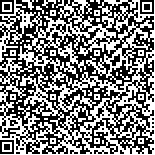
受高光谱成像仪低空间分辨率及复杂地物的影响,高光谱遥感图像存在大量混合像元。为提高地表分类精度以及满足亚像元级目标探测的需求,混合像元分解技术一直是高光谱遥感研究热点之一。本文主要对高光谱混合像元分解技术中的核心问题:端元数目估计、端元提取算法、丰度估计算法进行综述,系统地分析了各种典型算法的原理及优缺点,进一步阐述研究过程中建立高精度遥感混合反演模型与遥感产品业务化中的混合像元分解技术难题,同时针对今后混合像元分解技术发展方向,指出在继续引入新型算法理论方法基础上,结合用户应用需求,推进高光谱混合像元分解算法业务化应用,为高光谱遥感工程化应用提供支持。
Hyperspectral imaging, which measures electromagnetic energy scattered in the instantaneous field view in hundreds of bands, contributes significantly to earth observation. As the electromagnetic spectrum covers visible, near infrared, and shortwave infrared spectral bands, it holds abundant spectral information. The subtle discriminative spectral characteristics also make material identification possible. However, the signals recorded by low-resolution hyperspectral sensors from certain pixels under a complex background are a mixture of substances. To improve the accuracy of classification and subpixel object detection, Hyperspectral Unmixing (HU) represents an important solution, especially in the remote sensing area.Mixed pixels comprise a few materials called endmembers. The fraction of each endmember in a pixel is called abundance. Given the hyperspectral data exhibiting high dimension, special spectral correlation, and huge quantity, the determination of the number of endmembers can be regarded as dimensionality reduction. The objective of HU is to estimate and extract the spectral signatures and abundance of endmembers. In this study, mixing models are systematically discussed. On the basis of mixing models, the core problem of HU is presented, which mainly comprises three aspects: estimation of number of endmembers, endmember extraction, and abundance fraction estimation. For each aspect, we summarize the basic theory and development of the HU processing methods. Through the basic theory model, this work performs an initial classification of each aspect. The physical or mathematical problems involved are also discussed along with classical and state-of-the-art methods used to address the problems. Furthermore, a general overview of each category is provided, and a comprehensive analysis of the advantages and disadvantages of classic algorithms is performed. Through the comparison of different methods, this study offers a perspective on the potential and emerging challenges in the process of HU.The research on HU has been continuous. Even though extensive processing results have been achieved and numerous analyses have been performed, obstacles remain and call for solutions. Five challenges are identified in this work: curse of dimensionality, establishment of a high-accuracy mixed inversion model, endmember variation, establishment of remote sensing operational product, and development of algorithms for real-time processing. According to each challenge, the study performs further analysis and presents several remarks. Then, suggestions with regard to future research directions are offered.This study provides a brief overview of HU. With the development of hyperspectral imaging, the performance of imaging data has undergone a qualitative leap. However, different application requires different data types. That is, the most suitable data, and not the data with the highest index, should be adopted to solve the problem. Considering the future development trend of HU, this work suggests that new methods be combined with the demands of users to promote the operational application of HU and support hyperspectral remote sensing engineering applications.