下载中心
优秀审稿专家
优秀论文
相关链接
摘要
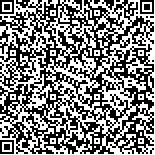
准确地获知灾区的建筑物损毁程度能为抗震救灾和灾后重建提供决策依据。利用震后极化合成孔径雷达(SAR)数据,该文提出了一种综合利用极化分解后多纹理特征的震后建筑物损毁评估方法。首先,用Pauli分解的π/4偶次散射分量剔除非建筑区;其次,用Pauli分解的π/4偶次散射分量的方差特征、对比度特征和Pauli分解的奇次散射分量的对比度特征识别倒塌建筑物,并分别基于区块计算建筑物损毁指数;最后,综合3个纹理特征完成建筑物的损毁评估。采用玉树震后RADARSAT-2数据和东日本大地震后ALOS-1数据的实验验证了所提方法对建筑物损毁评估的有效性,该方法对玉树城区和日本石卷城区的重度、中度和轻度损毁建筑评估的总体精度分别为74.39%和80.26%。与其他方法的对比实验表明,该方法能减少取向角的影响,对存留有少数与方位向平行的完好建筑物的倒塌区、大取向角的完好建筑区的评估更为准确。
Accurately obtaining building damage situation in disaster areas provides decision-making support for disaster relief and post-disaster reconstruction. The existing evaluation methods using polarization featuresare greatly influenced by the orientation angle of buildings, and the intact buildings with large orientation anglesare easily misclassified for the collapsed buildings. The combination of multiple features is an effective way to improve the accuracy of building damage assessment, in which the texture features play an important role.
This paper proposed a new building damage assessment method which applies the texture features of polarization decomposition components. There are three key procedures in this method. Firstly,the non-building areasare removed by thefiltered π/4 double-bounce scattering component of Pauli decomposition. Secondly,thestrategy of extracting collapsed buildings is built.For building areas, the Variance and Contrast features of Gray-Level Co-occurrence Matrix (GLCM) are calculated on the Pauli decomposition parameters. Specifically, the Variance and Contrast textures are obtained by the π/4 double-bounce scattering component of Pauli decomposition; the Variance texture is given by the odd scattering component of Pauli decomposition. Then, the appropriatethresholds for distinguishing collapsed buildings from intact buildings are determined by these texture features.When the texture feature value of the pixel is smaller than the threshold, then the pixel is classified as collapsed building, otherwise, the pixel is identified as intact building. At last,building damage assessment is implemented. The building damage indexes computed by the three texture features are averaged to get the final building damage index, and then the result of building damage assessment is obtained.
This method was validated on RADARSAT-2 fine-mode polarimetric SAR imagery from the Yushu earthquakeacquired on April 21, 2010. The ground-truth map of the buildings in Yushu city, interpreted on high-resolution optical data, was used as a reference for comparison. Compared with the H-α-ρ method, the method with homogeneous texture of span image and the method with integrated Normalized Circular-pol Correlation Coefficient (NCCC) and homogeneous texture feature, the building damage assessment accuracy of the proposed method was the highest, and its overall accuracy was improved to 74.39%.Particularly, the detection rate for serious damage buildings is 100%, and the false alarm rate is 15.62%. In addition, the intact buildings with large orientation angles in northeastern corner of the city were also extracted correctly. Based on the experimental analysis, the main factor of affecting the accuracy is the similarity of Pauli polarization features, the Variance and Contrast texture features betweenthe flat and dense intact building areas and serious damaged building areas. In addition, the effectiveness of the proposed method was verified by the ALOS-1 data after the 2011east Japan earthquake and tsunami, and its overall accuracy was improved to 80.26%.
A damage assessment method based on texture features from GLCM of Pauli decomposition components is proposed in this paper. In this method, Pauli decomposition parameter was utilized to remove non-building areas, which was capable of removing the non-building areaseffectively, such as rivers, roads, bare ground and so on. By the comprehensiveutilization of the GLCM texture features of three Pauli decomposition components, the collapsed buildings can be extracted more accurately. By comparison with other methods, the results confirm the validity of the proposed method.