下载中心
优秀审稿专家
优秀论文
相关链接
摘要
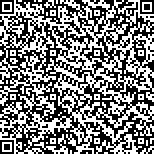
在多云多雨的地区,光学遥感存在着获取无云数据困难的难题,这会导致时间序列应用中可用数据匮乏。因此,本文面向稀疏时间序列遥感数据,根据噪声造成遥感影像上归一化差分植被指数(NDVI)被低估的事实,提出了一种知识引导的拟合方法。首先,在遥感影像预处理的基础上,利用先验知识和时序差分法对噪声进行识别和剔除;然后,采用高斯二阶模型对原始数据进行拟合;最后,根据拟合残差更新权重,进行迭代拟合,重复上述过程直至获得稳定的结果。本文以Landsat 8 OLI作为数据源,对浙江省杭州地区的森林数据进行拟合,结果表明:在稀疏时间序列数据的情况下,本文方法与MODIS数据拟合结果的相关系数达到0.92,关键时点(如NDVI峰值点等)的时间误差在5 d;相比当前主流方法的0.88与8 d具有更高的精度。
Time series remote sensing plays an important role in forest monitoring, and the performance of the fitting method of time series Normalized Difference Vegetation Index (NDVI) determines the precision of phenology parameter estimation. However, in cloudy and rainy areas, obtaining cloud free remote sensing data for time series application is greatly difficult, leading to the difficulty in precisely fitting the time series NDVI.
We present a knowledge-guided fitting method for sparse time series remote sensing data, given that the NDVI is underestimated by noise. First, we use prior knowledge and time difference method to identify and remove the noise, and then the Gaussian second-order model is used to fit the original data. Third, the iterative fitting is carried out by updating the weights of original data according to the fitting residuals. Finally, this process is repeated until a stable result is obtained.
We use NDVI derived from Landsat 8 OLI as the data source to fit the forest data in Hangzhou, Zhejiang province. MODIS data are used as the referenced data to test accuracy of fitting result. The correlation coefficient between the fitting result of sparse time series data and the MODIS data fitting results is 0.92, and the difference of the day of max NDVI is five days, which indicates the proposed can be used to estimate the growth intensity, growth period, and other biological parameters effectively, the result is closer to the MODIS data fitting result compared with the state of art method.
Our research provides the knowledge-guided fitting method, which is more effective than the other conventional methods, especially for the sparse time series data. This method reduces the human subjective influence and the excessive disturbance caused by the noise in the original data, making the fitting result more close to the actual fitting curve. The advantages of this method include less input parameters, fast converge, and the stability of fitting result. However, the proposed method suppresses the noise in the fitting processing to obtain relatively smooth fitting curve, which also may lead to the loss of the curve information; further improvements can be performed in the future.