下载中心
优秀审稿专家
优秀论文
相关链接
摘要
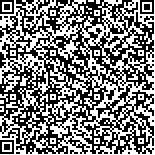
遥感识别多源特征综合和特征优选是提高遥感影像分类精度的关键技术。农作物遥感识别中,识别特征的相对单一和数量过多均会导致作物识别精度不理想。随机森林(random forests)采用分类与回归树(CART)算法来生成分类树,结合了bagging和随机选择特征变量的优点,是一种有效的分类方法。单变量特征选择(univariate feature selection)能够对每一个待分类的特征进行测试,衡量该特征和响应变量之间的关系,根据得分舍弃不好的特征,优选得到的特征用于分类。本文基于随机森林和单变量特征选择,利用多时相光谱信息、植被指数信息、纹理信息及波段差值信息,设计多组分类实验方案,对江苏省泗洪县的高分一号(GF-1)和环境一号(HJ-1A)影像进行分类研究,旨在选择最佳的分类方案对实验区主要农作物进行识别和提取。实验结果表明:(1)多源信息综合的农作物分类精度明显高于单一的原始光谱特征分类,说明不同类型特征的引入能改善分类效果;(2)基于单变量特征选择算法的优选特征分类效果最佳,总体精度97.07%,Kappa系数0.96,表明了特征优选在降低维度的同时,也保证了较高的分类精度。随机森林和单变量特征选择结合的方法可以提高遥感影像的分类精度,为农作物的识别和提取研究提供了有效的方法。
Timely accurate crop type identification and Crop Acreage Estimates (CAE) are essential for food security. Remote sensing technology has been successfully applied to crop identification because of its macro, rapid monitoring capabilities at large scales and its ability to quickly obtain accurate agricultural information. However, when identifying crop types, both simple and too many identifiable features might lead to low classification accuracies. Thus, multi-source and optimally selected features are obviously crucial to crop classification using remotely-sensed images. This paper considered a series of features, including multi-temporal spectra, vegetation indexes, textures, and band differences. Multiple experiments were designed and conducted in Sihong County, Jiangsu Province, China using Gaofen-1 and Huanjing-1 images to evaluate the influence of different features on the identification accuracy and determine the combination of preferred features which can improve the classification effect. The combination of random forest classification and univariate feature selection methods was expected to have a considerably positive effect on distinguishing and extracting the main crops in remote sensing images.
In this study, the crop classification was implemented using random forests and univariate feature selection. The random forest method, which constructs many CART decision trees during each classification process, is one of themost effective classification methods. Univariate feature selection is a statistical testing method, which tests each feature to measure the relationship between the feature and the corresponding variable and then removes features that obtain low scores. First, the random forest classifier was applied to classify the images using the preceding multisource features mentioned. Second, we analyzed the contributions of different types of features or feature combinations to the classification accuracy. Third, features were selected by using the univariate feature selection method. Finally, we re-combined the optimal features and random forest to classify the image and distinguish the main crop types with high accuracy.
The results showed that overall classification accuracy based on the combination of optimal features reached 97.07% with the corresponding Kappa coefficient being 0.96, which indicated that the feature selection method used in this paper has a considerably positive effect on high classification accuracy because it efficiently reduced feature dimension. The classification results also showed that the crop classification using multi-source features outperformed the one which only used spectral features. In addition, the accuracy of the experiment which simultaneously used spectral and VI features was the second highest among all experiments. The optimal feature combination has 19 features, including five spectral features, six vegetation indexes, seven band difference features, and 1 texture feature, which suggested that vegetation indexes and band differences were more important to the crop identification than the other two.
This study demonstrated the following: (1) the addition of different types of features could improve classification accuracy; (2) too many features would decrease classification accuracies; (3) univariate feature selection was effective for choosing the optimal subset of features. The optimally selected features can be relatively beneficial to reduce the computation load and improve the worse accuracies caused by applied features blindly. Therefore, the combination of random forest and univariate feature selection is effective in improving classification accuracy and efficiency.