下载中心
优秀审稿专家
优秀论文
相关链接
摘要
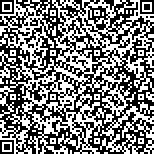
合成孔径雷达(SAR)稀疏成像模型中的参数选择对于SAR稀疏成像的性能有重要影响,也是当前SAR稀疏成像研究中的难点问题。已有参数选择方法普遍存在适用于个别模型或者运算量大的缺点。基于最大后验概率估计和贝叶斯推理,提出了一种无需额外先验信息的自适应参数选择方法,所有需要的参数都可从已知的数据中获取。通过推导得到模型参数与信号、噪声方差的关系,避免了对数据进行一系列的训练处理,因此极大地减小了计算量。仿真数据和实测数据处理表明,本文方法在实现了较为精确的参数优化选择的前提下,其计算量远低于贝叶斯信息论准则、L-曲线等已有参数选择方法。
High resolution is an important direction for the development of Synthetic Aperture Radar (SAR).Conventional SAR imaging algorithms based on matched filtering is limited by the signal bandwidth and the synthetic aperture length.Compressive Sensing (CS) theory has recently attracted the attention of domestic and foreign scholars.Electromagnetic scattering signals of man-made objects,such as vehicles and buildings,have obvious sparse characteristics,and the total electromagnetic scattering of the target can be approximated by the synthesis of the center scattering of the local scattering.Studies have shown that CS has considerable potential in improving the quality of radar imaging and high precision imaging of man-made targets.
However,the application of CS theory in radar imaging is novel;therefore,it has some problems that require further study.Studies have shown that the regularization and shrinkage parameter have an obvious impact on the performance of radar sparse imaging.Traditional parameter selection methods have serious disadvantages in computation cost and accuracy or are limited to a few specific models.Therefore, proposing a parameter selection method is of great importance.
A fully automated algorithm is proposed in this paper to improve the performance of radar sparse imaging in the case of parameter optimization and to overcome the preceding disadvantages mentioned.First,combined with the Maximum A Posteriori (MAP) estimation and Bayesian inference,we obtain the relationship between regularization and shrinkage parameter.The value of regularization parameter is determined by the choices of shrinkage parameter,noise,and signal variance,thereby reducing the dimension of the parameter selection problem.Second,we propose a radar sparse imaging algorithm which can solve the joint optimization problem to simultaneously achieve model parameters estimation and SAR imaging.In each iterative of the approach,all required parameters are updated with training data without the necessary prior information,and the image is reconstructed by regularization technique with the updated observation model constructed by new parameters.
Extensive comparisons are carried out between the proposed method and several other competing methods based on simulations and real-data processes.The experimental results demonstrate that the proposed method can achieve accurate parameter estimation and imaging performance with low computational complexity.Compared with the traditional imaging method,the imaging results of this method are sparse,thereby reducing the sidelobes and maintaining cleanliness without noise.
This paper presents a fully automated parameter selection method based on maximum a posteriori estimation and Bayesian inference without extra prior information.All required parameters can be obtained through known data.We deduce the relationship between model parameters and signal and noise variance;thus,a series of training processes has been avoided,and the computational cost has been considerably reduced.Simulations and realdata experiment demonstrate that the proposed method can achieve accurate parameter selection with lower computational cost than other parameter selection methods,such as Bayesian information criteria and L-curve.