下载中心
优秀审稿专家
优秀论文
相关链接
摘要
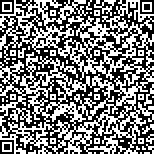
2. 中南大学 空间信息技术与可持续发展研究中心, 长沙 410083
以洞庭湖流域为研究区,对大范围湿地信息遥感提取方法进行了研究。先基于时间序列MODIS EVI及物候特征参数,通过J-M(Jeffries-Matusita distance)距离分析,构建了MODIS(250 m)最佳时序组合分类数据;其次,通过Johnson指数确定了最佳分割尺度,采用面向对象的遥感分类方法(Random tree分类器)提取了洞庭湖流域的湿地信息,并验证该方法的适用性。研究结果表明,基于时序数据与面向对象的Random tree分类的总体精度和Kappa系数分别为78.84%和0.71,较之基于像元的相同算法的总体分类精度和Kappa系数分别提高了5.79%和0.04。同时,基于面向对象方法的湿地整体的用户精度与生产者精度较基于像元方法分别提高了4.56%和6.21%,可有效提高大区域湿地信息提取的精度。
The mapping of large-scale wetlands involves time-series coarse spatial resolution remote sensing data and pixel-based methods, such as the decision tree and threshold techniques. However, few studies use low spatial-resolution images(such as moderate-resolution imaging spectroradiometer (MODIS)) and object-oriented methods to extract information from large-area wetlands. Although spatial resolution has some disadvantages, the coarse spatial resolution image has high time resolution, considerable spectral information, and low cost. Therefore, the high temporal characteristics of coarse image and object-oriented method can be used to extract wetland information over a large area, such as basins and continents.
In this study, the object-oriented method and time-series MODIS Enhanced Vegetation Index (EVI) data are utilized to map the wetland of the Dongting Lake Basin. The time-series MODIS EVI images are smoothed using the double logistic function fitting method of TIMESAT software package, which is based on MATLAB. Meanwhile, the phenology indices are calculated from the time-series MODIS EVI data. Subsequently, the best combination of images and optimal segmentation scale are determined with the JBh distance and Johnson index. Wetland mapping is then verified using a random tree classifier based on the segmented images. In addition, validation data are derived from the visual interpretation of Landsat 8 images, Google Earth, and land-use data. To verify the classification effect of the object-oriented classification method on coarse spatial resolution images, the pixel-based method is also utilized to classify the best combination of images and is then compared with the upper method.
The phenology of various ground cover types is obviously different, which indicates that they can be used to distinguish different land types, especially vegetation types. Given the image combination of the critical periods (DOY113, DOY145, DOY173, DOY193, DOY241, and DOY289) of vegetation growth and phenology (start of season and length of season), we can determine the JBh(9.143) and JM distances to meet research needs. An optimal segmentation scale parameter (15 pixels of MODIS) is obtained using the Johnson index. Based on object-oriented classification method, the overall accuracy and Kappa coefficient of the random tree classifier are 78.84% and 0.71 respectively. Compared with the object-oriented method, a pixel-based classification method with random tree classifier achieves a lower overall accuracy and Kappa coefficient of 73.05% and 0.67, respectively. In traditional pixel-based analysis, the surrounding pixels contribute a substantial proportion of signals. The object-oriented method analysis utilizes objects instead of pixels, which effectively reduces signals from surrounding pixels by integrating neighborhood information. The object-oriented classification method also reduces the "saltand pepper" effect of mapping heterogeneous landscapes and enhances the accuracy of the analysis. However, large pixels of water still exist, thus causing existing mutual fault points (368 and 228). A total of 237 and 316 pixels of sedge and reed are classified to water and 113 and 128 pixels are classified to mudflat. However, 683 and 502 pixels of paddy are misclassified into dry land and forest. We also obtained higher user accuracy for the whole wetland through the object-oriented classification technique than pixel-based classification method. Both the user and producer accuracies improved to approximately 4.56% and 6.21%, respectively. The user and producer accuracy of wetland categories were approximately 74.75%-88.03% and 78.68%-84.36%, respectively, based on the object-oriented method, which considerably increased compared with pixel-based method except for the user accuracy of the sedge wetland. On the one hand, this finding can be attributed to strong heterogeneity and mixed pixels. On the other hand, the influence of human activity, disturbance, crop prices, and national policy caused an increasingly broken patch of sedge field, thus causing misclassification.
The combination of time-series MODIS EVI data and object-oriented method effectively extract wetland information on a watershed scale. It provides a new method and technique for wetland mapping on a large scale, even in a continental range.