下载中心
优秀审稿专家
优秀论文
相关链接
摘要
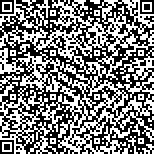
航空热红外遥感影像的航带宽度一般较为有限,通常需要进行连续飞行获得多个航带才能覆盖一个较大的研究区;由于地表温度随时间变化迅速,不同航带间地表温度存在差异。因此,进行不同航带影像拼接之前,需要对不同航带的地表温度进行时间归一化。本文基于温度日变化模型,构建了航空遥感地表温度时间归一化方法;结合HiWATER试验区高密度的气象观测数据,分析了天气晴朗条件下,风速的大小和波动剧烈程度对地表温度日变化模型的影响,在此基础上发展了一种考虑风速影响的改进温度日变化模型。验证结果表明:两种模型均能够减小观测时间不同导致的地表温度瞬时波动差异;考虑风速影响的改进模型比未改进模型的精度提高0.3-0.6 K,且其提供的地表温度在时间尺度变化上更加符合实际情况。本研究建立的相关模型对于卫星遥感地表温度的时间归一化也具有借鉴意义。
Airborne thermal infrared remote sensing can obtain Land Surface Temperature (LST) data at a high spatial resolution. However, the swath width of airborne stripes is commonly limited, and researchers must generally acquire multiple stripes of continuous flights to cover a larger study area. LST changes rapidly over time, and the temperature clearly varies among different stripes. Thus, the temporal normalization of LST is necessary to obtain a large scene mosaicking image of different airborne stripes. However, studies on the temporal normalization of airborne LST are still rare to date.
The present study first developed a practical method based on the Diurnal Temperature Cycle (DTC) model to temporally normalize the airborne LSTs in cloud-free skies. We assumed that two factors (i.e., the typical diurnal pattern and instantaneous fluctuation from the mean atmospheric conditions) were involved in detecting LST. We then established an Improved DTC (IDTC) model that analyzes the influences of wind speed and fluctuations that cannot be measured by the DTC model. The temporal normalization of the LST requires two parts. The first aspect is the DTC variables, including Ta, tm, ts, and δT. Each land cover type has its unique DTC pattern because of its physical characteristics. Therefore, in-situ LSTs in a diurnal cycle at each ground site were employed to calculate the DTC variables through the Levenberg-Marquardt method. A look-up table (LUT) for the DTC variables of different land cover types was then constructed. The linear regression technique was subsequently utilized to evaluate the LST instantaneous fluctuation of the second part. The TASI stripes were checked pixel by pixel. The variables for each pixel were then searched from the LUT to normalize each TASI stripe.
First, the DTC and IDTC models were evaluated in the temporal normalization of the TASI LST on July 10, 2012 by selecting the Heihe Watershed Allied Telemetry Experimental Research (HiWATER) experiment region as the study area. Results show that the TASI LST normalized by the IDTC model was generally more consistent with the ground measured LST than the DTC model. The accuracy of the IDTC model was approximately 0.3 K to 0.6 K higher than the DTC model. Second, to better demonstrate the performance of the IDTC model in the entire study area, the TASI LST images that had been normalized to the ASTER overpass time (12:12 local time) were up-scaled to 90 m and then compared with the ASTER LST. The data indicate that both the temporal normalization methods (DTC and IDTC models) could decrease the LST differences among different TASI stripes. The TASI LST normalized by the IDTC model was more consistent with the ASTER LST, especially in the Gobi Desert and desert steppe. Third, the temporal normalization of the ground measured LST was also implemented to test the IDTC model. It further demonstrates that the IDTC model was better than the DTC model in the temporal normalization of airborne LST.
This study proposes a practical method that temporally normalizes the airborne LST based on the DTC and IDTC models to lower the temperature difference among different stripes. Results indicate that the temporal normalization based on the DTC and IDTC models can effectively obtain a large scene composed of multiple flight lines before mosaicking different stripes. The IDTC model, which involves the influences of wind speed and LST fluctuation, also performs better than the DTC model. Our present research has presented a new perspective on the temporal normalization of satellite LST.