下载中心
优秀审稿专家
优秀论文
相关链接
摘要
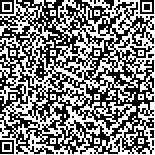
双线性混合模型是近年来非线性光谱解混的研究重点之一,其克服了线性混合模型无法描述地物多重散射作用的缺陷,能够更精确地还原真实的地物光谱混合过程。然而,限于模型的复杂性,目前在缺乏准确的端元先验知识的条件下进行双线性光谱解混仍是一项具有挑战性的任务。差分进化算法(DE)是一种具有良好全局搜索能力的群智能优化算法,其优化求解过程无需进行复杂的数学推导,为双线性光谱解混问题提供了一种有效的解决途径。为此,本文以FAN双线性混合模型为例,提出了一种双种群机制的差分进化算法(记为DE-FAN),实现非监督双线性光谱解混。DE-FAN算法通过建立端元与丰度两个种群的交替进化机制寻找最优解,同时在迭代中引入自适应重构策略增强种群多样性,降低算法陷入局部最优解的风险,最终实现端元与丰度的同时估计。通过模拟图像及真实图像的解混实验进行算法检验,证明DE-FAN算法较之传统非线性解混算法具有更高的解混精度及解混效率。
Hyperspectral unmixing plays an important role in remote sensing applications by extracting the pure constituent spectra and correspondent fractions in mixing pixels. Bilinear mixing models have recently become a hot topic in nonlinear spectral unmixing research. Nevertheless, most of the existing bilinear unmixing algorithms require prior knowledge about the endmembers, which can be regarded as supervised algorithms. This paper reports on a new unsupervised unmixing algorithm based on the FAN bilinear mixing model by employing the differential evolution (DE) algorithm (i.e., DE-FAN), which can solve the bilinear unmixing problems more efficiently and accurately compare with existing unsupervised bilinear unmixing algorithms.Considering the bilinear unmixing problem with hyperspectral imagery, the proposed algorithm makes the following improvements on the standard DE algorithm. First, the mutation factor is tuned dynamically rather than fixed during the iterative process, which can significantly enhance the neighborhood search capability. Second, considering the acceleration of the convergence rate, DE-FAN constructs a double-population (i.e., endmember population and abundance population) framework such that an alternative evolution procedure is presented. Third, the population re-initialization tactic is introduced to enhance the capability of large-scale optimization. It can decrease the number of required population individuals, thus improving the computational efficiency. It can also significantly lower the trapping risk in local optimums. Finally, a cooperative co-evolution tactic is considered. The hyperspectral image is divided into several sub-images and then processed by the DE-FAN algorithm separately. The final solution of the endmember estimation can be obtained by averaging the best solutions from the sub-images. The remaining iterations can continue by fixing the endmember variables with the final endmember solution. The final solution of abundance estimation is achieved when the stopping criteria is met.
We validate the DE-FAN algorithm by adopting synthetic datasets and real airborne visible/infrared imaging spectrometer images. Different endmember numbers, signal-to-noise ratios, and maximum abundances are also considered. Several state-of-the-art algorithms (i.e., MVC-NMF, SISAL+FCLS, MU-LQM, N-FINDR+CNLS, geodesic simplex volume maximization, and PG-FAN) are compared. Experimental results conducted utilizing both synthetic and real datasets indicate that the proposed DE-FAN algorithm outperforms other algorithms by obtaining more accurate endmembers and abundance.The DE-FAN algorithm overcomes the LMM shortcomings and solves the bilinear unmixing problem. The unmixing experiments demonstrate that DE-FAN can obtain more accurate results than other classical unmixing algorithms. We will consider the influence of the penalty coefficient and attempt an adaptive method in future work. We will also extend our proposed DE-FAN to more bilinear models such as the polynomial post-nonlinear model and generalized bilinear model.