下载中心
优秀审稿专家
优秀论文
相关链接
摘要
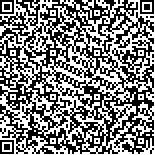
积雪是冰冻圈的重要组成部分,因其在可见光波段的高反射率、低导热率的特性以及大面积的覆盖,成为全球辐射平衡的重要决定因子。在中纬度的干旱和半干旱山区,季节性的冰雪融水是春季河川径流的主要补给水源,山区积雪分布的变化对融雪期河流径流量的波动具有重要影响。当前的积雪产品在下垫面为山区林地时会低估积雪面积,从而影响了山区水文过程模拟的精度。本文基于Landsat OLI影像,采用归一化差值积雪指数NDSI和归一化差值林地积雪指数NDFSI相结合的方法,对春季融雪期的阿尔泰山区泰加林地进行积雪识别,并采用海拔高度、温度、以及对应的高分数据对提取结果进行了定量分析。结果表明,采用NDSI进行积雪识别时,山区林地的积雪会被大量漏分;对林地像元采用NDFSI阈值法可以区分林地中是否有积雪分布。NDSI和NDFSI相结合的积雪识别方法操作简单,不需要提供森林分布图等辅助数据,可以有效提高山区林地复杂环境下积雪制图的精度。
Snow is one of the important parts of the crysphere, because its high reflectivity and low thermal conductivity can directly affect the ground and air temperatures, albedo of the Earth's surface, and soil moisture. In mid-latitude arid and semi-arid mountainous regions, seasonal melt are mainly supplies the spring runoff. The changes of snow distribution in mountainous regions exert an important influence on the fluctuation in river runoffs during the snowmelt season. The currently used snow products in mountainous and forested regions exhibit low accuracy when simulating mountainous hydrological processes. Thus, this research aims to develop a highly accurate snow cover mapping method for complex environments, such as mountainous and forested regions.
Normalized Difference Snow Index (NDSI) based on the spectral characteristics of snow is extensively used in mapping snow cover at the global scale. However, NDSI presents a small value and discrete distribution in snow-covered forests. Thus, it cannot effectively identify snow in forested areas. Meanwhile, Normalized Difference Forest Snow Index (NDFSI) is based on the combination of the spectral features of snow and forest and defined as NDFSI=(ρnir-ρswir)/(ρnir+ρswir). In this study, a snow-cover mapping method that combines the NDSI and NDFSI was used for snow extraction in the taiga forest of Altay Mountains from the Landsat OLI image acquired in spring. First, the NDSI was used in all the pixels. The pixels with NDSI of > 0.4 and ρnir of > 0.11 were classified as snow, whereas those with NDSI of > 0.4 but ρnir of ≤ 0.11 were classified as water. In this step, most snow without shielding can be recognized, and forest snow is usually not recognized because of the shielding by the forest crown. Second, NDFSI was used in pixels with NDSI of ≤ 0.4, and the pixels with NDFSI exceeding 0.4 were classified as forest with snow. Pixels with NDFSI of less than 0.4 were defined as forest without snow.
The snow extraction result shows that snow in the no-forest region can be extracted using a reasonable NDSI threshold value. However, the snow in the forested region cannot be recognized with NDSI alone. In NDSI, the snow-covered area is underestimated in the forested-region. Thus, NDSI and NDFSI were combined, and the snow extraction result was evaluated using DEM data, temperature inversion result, and GF-1 image. Result showed that the average altitude of the forest with snow is 1611 m, and the average altitude of the forest without snow is 1278 m. The reason is that snow in lower altitudes melts earlier than snow in higher altitudes during the snowmelt season. The average temperature of forest with snow and forest without snow are 6.8℃ and 15.3℃, respectively. These temperatures are consistent with those obtained from the field. In the GF-1 image with 2 m spatial resolution can indicate the presence of snow in a forest. According to the obtained GF-1 image, the snow extraction in this study is highly accurate, and thus most snow in forest can be extracted correctly.
NDFSI outperforms NDSI in the extraction of snow in forested areas. The accuracy of snow-cover mapping in complex mountainous and forested environments can be improved considerably by combining NDSI and NDFSI. Moreover, this approach can be applied easily without using other auxiliary data, such as forest maps.