下载中心
优秀审稿专家
优秀论文
相关链接
摘要
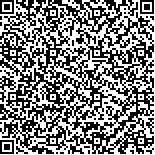
植被覆盖变化是生态环境领域的核心研究内容之一,但其估算精度常受到地形效应、土壤背景、大气效应等各种因素影响。以Landsat 8 OLI为遥感数据源,基于像元二分模型,分别利用归一化差值植被指数(NDVI)、经Cosine-C校正的归一化差值植被指数(NDVI)和归一化差值山地植被指数(NDMVI)建立植被覆盖度估算模型,以评估南方丘陵区植被覆盖度的地形效应。结果表明,3种植被覆盖度估算模型均能削弱地形效应,但消除或抑制地形效应影响的能力不同。比较而言,基于NDMVI指数构建的植被覆盖度估算模型的地形效应最小,更适合地形复杂区域的植被覆盖度遥感估算;基于Cosine-C校正的NDVI植被指数构建的植被覆盖度估算模型的地形效应次之,但存在一定的过度校正现象;基于NDVI植被指数构建的植被覆盖度估算模型的地形效应最大,尤其当坡度≥10°时,阴坡植被覆盖度比阳坡明显偏低。
关键词:
植被覆盖度 NDVI NDMVI Cosine-C校正 地形效应Changes in Fractional Vegetation Cover (FVC) is one of the core research fields in eco-environment assessment. FVC estimation from satellite image is significantly influenced by the terrain, soil, and atmosphere. Among of them, terrain variation can arouse miscalculation of biomass information of similar kind of vegetation, and it is a main bottleneck of quantitative remote sensing of vegetation. Therefore, it is urgent to find a method of FVC estimation that can eliminate or reduce the effects of terrain variation, in order to improve the FVC estimation using the satellite data over mountainous and hilly areas.
Taking Landsat 8 OLI image as a data source. Firstly, three vegetation indexes, including the normalized difference vegetation index (NDVI), Cosine-C corrected NDVI, and the normalized difference mountain vegetation Index (NDMVI), are estimated. Then FVC are calculated from three vegetation indexes, using a linear unmixing model. Finally, the impact of terrain variation of three estimation methods of FVC are assessed and compared, considering altitude, slope, aspect, and solar incident angle. five altitude ranges, (i.e. 100-400 m, 400-600 m, 600-800 m, 800-1000 m and ≥1000 m), 72 aspect ranges (from 0 to 360° with a 5° interval), four slope ranges (i.e. 0-10°, 10°-20°, 20°-30° and ≥30°), and ten solar incident angle ranges (i.e. 0-10°, 10°-20°, …, ≥90°) are considered, and the Coefficient of Variation (CV) is used to measure the impacts.
The results show that the CV is minimal when the altitude is lower than 400 m, and the CV increases with the increasing altitude in three method. The minimal CV is 0.39%, -8.15% and -3.14% for NDVI-based method, NDVI-C based method and NDMVI-based method, respectively. The CV also increases with increasing slope. The CV of NDVI-based method reaches 11.5% when the slope is larger than 30°, indicating a significant impact of terrain variation. The CV of NDMVI-based method is slightly larger than that of NDVI-C based method when the slope is lower than 10°. The CV of NDMVI is the nearest to 0 when slope ranges from 20° to 30°, indicating a little impact of terrain variation.
The terrain effects are reduced for all three VI-based methods. The NDMVI-based method has a best performance, and the terrain variation has little effect on FVC estimate. However, there is over-corrected phenomenon when the Cosine-C is corrected. The NDVI-based method has the poorest result, indicating that it is heavily influenced by the terrain variation when the slope is larger than 10°. The estimated FVC in shady slope is significantly lower than that of sunny slope.
Based on the analysis above, it is shown that the NDMVI-based method can effectively eliminate or reduce the effects of terrain variation to improve the FVC estimation from the remote sensing data over mountainous and hilly areas in southern China. However, only Landsat 8 image with spatial resolution of 30 m is employed in this study. The magnitude of terrain effects may also depends on the spatial resolution of image. Therefore, further studies should be focused on terrain correction for high spatial resolution satellite image.