下载中心
优秀审稿专家
优秀论文
相关链接
摘要
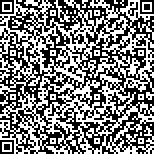
传统的多时相遥感图像分类大多拘泥于单一特征,本文基于多时相的Landsat 8遥感数据,开展了综合多特征的特征提取与特征选择方法研究。综合了NDVI时间序列、最佳时相反射率光谱特征以及纹理特征作为初始分类特征,并采用基于属性重要度的粗糙集特征选择算法对其进行特征约简。分类结果表明:(1)利用初始分类特征,分类的总体精度达到92.81%,棉花提取精度达87.4%,与仅利用NDVI时间序列相比,精度分别提高5.53%和5.05%;(2)利用粗糙集选择后的特征分类,分类总体精度可达93.66%,棉花分类精度达92.73%,与初始分类特征提取结果相比,棉花分类精度提高5.33%。基于属性重要度的粗糙集特征选择不仅提高了分类精度,同时有效降低了分类器的计算复杂度。
关键词:
多时相Landsat 8数据 综合多特征 NDVI 棉花提取 特征选择Cotton is a significant economic crop, and cotton extraction plays an important role in effective and controllable agricultural management. Multi-temporal remote sensing images have been widely used in cotton extraction, but these studies mainly focused on sole features, such as the Normalized Difference Vegetation Index (NDVI). An effective method of integrated multi-features based on multi-temporal Landsat 8 images was proposed to extract cotton information.
In this study, we chose north-central Shawan County in Xinjiang Uygur Autonomous Region as the study area. Nine images taken by Landsat 8 in 2013 were collected for cotton extraction. NDVI time series were generated to characterize the phenological pattern of each land cover type. The optimal temporal reflectance image was selectedby analyzing the difference in NDVI profile between cotton and other crop types. Texture features were calculated by the gray-level co-occurrence matrix method. NDVI time series, optimal temporal reflectance image, and texture features were combined as the original classification features. When the training samples were sufficient,butthe featureswereexcessive, the classification accuracy may decrease because of redundant information. We completed feature selection by using the rough set method and then obtained the selective features of the original features. The NDVI time series, original features, and selective features were used for classification by the support vector machine. The cotton distribution map was generated based on the classification result of the highest accuracy. Finally, we evaluated the accuracy of classification results by confusion matrix.
The selection of the optimal temporal reflectance image for cotton identification is important, and the optimum phase of this studyis on September 4. In this period, wheat was harvested; corn and sunflower were in the mature period; andcotton was in the blossom period. Significant differences were observed among these crops in the optimum phase. The original features achieved accuracies of 87.4% and 87.93% for cotton producers and users, respectively, and the overall accuracy was 92.81%. Compared with the classification results of the NDVI time series, the overall accuracy increased by 5.53% and the accuracy of cotton producers increased by 5.05%.Moreover, the classification accuracies of other land cover types increased to varying extents. The selective features achieved accuracies of 92.73% and 90.36% for cotton producers and users, respectively, and the overall accuracy was 93.66%. Compared with the classification results of the original features, the overall accuracy increased by 0.85% and the accuracy of cotton producers increased by 5.33%.
Experiments showed that feature selection by the rough set method not only improved the classification accuracy but also effectively reduced the classification complexity. The proposed method achieved an accuracy of 92.73% for cotton extraction. The method of integrated multi-features based on multi-temporal Landsat 8 images is promising for crop extraction, even for land cover classification.