下载中心
优秀审稿专家
优秀论文
相关链接
摘要
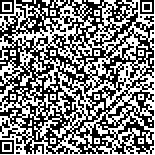
确定图像类别数是图像分割中的重要任务,在大多数分割算法中需由用户预先指定类别数。受地物目标及其分布的多样性、复杂性和未知性等因素的限制、对彩色遥感图像而言,人为确定其类别数非常困难。为此,提出了一种基于区域和统计的可变类分割方法,融合规则划分技术和RJMCMC算法,利用规则划分将图像域划分成若干个规则子块,并假设每个规则子块内的像素服从同一独立的多值Gaussian分布;在此基础上由贝叶斯定理构建图像分割模型;利用Reversible Jump Markov Chain Monte Carlo(RJMCMC)算法模拟该模型,实现图像类别数的自动确定及图像粗分割;为了进一步提高图像分割精度,设计精细化操作,对Worldview-2合成及彩色遥感图像和多光谱IKONOS图像进行可变类分割,实验结果表明,提出方法不仅能自动确定图像类别数,还可以较好地实现区域分割。本文方法较好地实现彩色遥感图像的可变类分割。
Image segmentation has been a hot topic in image processing. It involves two tasks:determining the number of homogeneous regions and segmenting them. Most image segmentation algorithms mainly focus on the latter and determine a priori the number. Artificially determining the number of classes is difficult for certain reasons. Consequently, the number should be automatically determined.
A statistical and region-based segmentation approach for color remote-sensing images is introduced in this paper. First, the image domain is partitioned into groups of regular sub-regions (or blocks) by regular tessellation. Second, the image is modeled on the assumption that the intensities of its pixels in each homogeneous region follow an identical and independent multivariate Gaussian distribution. The Bayesian paradigm is applied to establish the image segmentation model. Third, a reversible-jump Markov chain Monte Carlo (RJMCMC) scheme is designed to simulate the segmentation model, which determines the number of classes and roughly segments the image. A refined operation is performed to improve the accuracy of the image segmentation results further.
Real and synthetic color remote-sensing images from Worldview-II and multispectral IKONOS images are tested. Qualitative and quantitative evaluation results are obtained to verify the feasibility and effectiveness of the proposed method. The proposed method exhibits advantages over two other segmentation methods, namely, the ISODATA method and the segmentation method combining Voronoi tessellation with EM/MPM algorithm.
An image segmentation approach based on regular tessellation and RJMCMC algorithm is proposed in this study. The proposed approach can not only automatically determine the number of classes but also segment homogenous regions better. Furthermore, the test results also show that the approach demonstrates high performance and high efficiency. In future studies, other tessellation methods can be used to partition the image domain.