下载中心
优秀审稿专家
优秀论文
相关链接
摘要
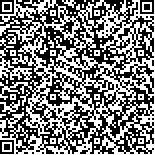
Landsat 8 OLI影像已成为重要的数据源,但受云及云影的影响较大,降低了数据的可用性,因此,快速识别云及云影,为后续的数据恢复有着积极的应用价值。通过构建云指数(CI)、归一化暗像元指数(NDPI)和比值阴影指数(RSI),采用阈值法和方位角搜索法,以两景Landsat 8 OLI影像(一景试验影像,另一景验证影像)为例进行云及云影检测。每类随机选取200个样点进行精度分析,结果表明:CI可快速区分OLI影像中的云区与非云区,厚云样本点正确识别率达到99%;NDPI与归一化植被指数(NDVI)构建的比值阴影指数RSI放大了水体、云影与其他阴影间的差异,更便于区分;方位搜索合理设置搜索方位角和搜索距离,简化了云影与云的相对关系模型,可准确区分水体与云影,两者的正确识别率都超过93%,弥补了阈值法的局限性。本方法可行快捷,为OLI影像的后续应用提供了基础,可有效提高其利用精度。
Landsat 8 OLI images have become important data sources; however, they are usually covered by clouds and cloud shadows, which reduce data availability. Therefore, a rapid method for detecting clouds and cloud shadows in a single image is necessary for follow-up data recovery applications. Threshold setting has been a commonly used method; however, it is difficult to use because the same threshold value usually indicates different objectives and varies across different data sources, as well as across different period images. Thus, the relative position relationship between a cloud shadow and a cloud is the key to detect a cloud shadow. First, a cloud index (CI) with a cirrus band and coastal/aerosol band was established to distinguish the thin and thick cloud pixels by setting thresholds. Second, a normalized dark pixel index (NDPI) with coastal/aerosol band and short-wave infrared 2 band was established. Furthermore, a ratio of shadow index (RSI) was established based on the NDPI and normalized differential vegetation index. RSI was used to distinguish the potential cloud shadow pixels in thin-cloud and non-cloud areas also by using thresholds. Third, an azimuth search method based on the solar azimuth angle and an appropriate searching distance were adopted to detect the cloud shadow from the potential cloud shadow pixels in the images. Two Landsat 8 OLI images were selected for the case study; the image captured on April 22, 2014 was used for the test, whereas the image captured on July 12, 2015 was used for validation. For each type (e.g., thick cloud, water, cloud shadow, or other kinds of shadow),200 random sampling points were used to assess the detection accuracy. Results showed that CI could quickly distinguish cloud from on-cloud pixels by statistics according to the cloud coverage ratio in the header files, with thresholds of CI ≥ 0.0011 and CI ≥ 0.0048 in the test and validation OLI images for the thick cloud type. Both user accuracy rates of detection for the thick cloud samples were over 99%. RSI could enhance the difference among water, cloud shadow, and other kinds of shadow, thereby facilitating the differentiation among the different types. The pixels with 0.45 ≤ RSI < 0.76 in the test image and 0.36 ≤ RSI < 0.76 in the validation image belong to potential cloud shadows. Using the solar azimuth angle at the time of imaging as the searching azimuth angle and a reasonable searching distance (500-2200 m in the test image and 270-800 m in the validation image), the azimuth searching method simplified the models of the relative position relationship of a cloud shadow to a cloud and accurately distinguished cloud shadow from water and other shadows. The detection precision levels for both test and validation reached more than 93%, which compensated for the limitation of the threshold method. The proposed shadow detection method combines threshold with simplified relative relationship model and leverages band difference. The method is feasible and rapid when applied to a single image, further improving the utilization accuracy of OLI images.