下载中心
优秀审稿专家
优秀论文
相关链接
摘要
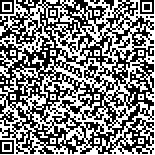
针对遥感图像的“时空矛盾”,评述了当前解决这一问题最主要的方法即遥感时空信息融合的方法,包括基于变化模型的融合、基于重建模型的融合以及基于学习模型的融合。通过分析各个模型的研究现状,指出了每种模型方法的优劣,特别重点介绍了影响较大的自适应时空融合方法的理论以及对其的改进算法。同时本文总结了当前时空融合模型在长时间序列模拟以及大区域数据集生成等方面的实际应用的效果,以及分析了影响时空融合结果的主要因素。最后基于这些问题和影响因素提出了今后时空融合模型发展的目标和方向。
Remote sensing images can provide important and abundant information about the Earth at a global or local scale. Thus, many applications often require remote sensing data with high acquisition frequency and high spatial resolution. However, meeting this requirement is a considerable challenge given satellite limitations. The spatiotemporal fusion method provides a feasible way to solve these "spatialtemporal" contradictions.
In the last 10 years, spatiotemporal fusion has elicited wide interest in various applications because it integrates the superiority of multisource satellite data in fine spatial resolution or frequent temporal coverage and it can generate fused images with high spatial and temporal resolution. In this study, we reviewed the advantages and limitations of three types of method for spatiotemporal fusion, namely, transformation-based, reconstruction-based, and learning-based methods.
First, the transformation-based method consistently filters and processes transformed data and then accesses high-spatiotemporal resolution data via inverse transform. It mainly focuses on the spatial and spectral information of multi-source satellite image enhancement or fusion. The spatial resolution of the results obtained with this method remains low, and the accuracy is relatively poor because the temporal change information is not used in this method. Second, the reconstruction-based method has elicited much attention since the proposal of a semi-physical fusion model and STARFM. This method integrates the information of temporal change, spatial change, and spectral change among multi-source satellite images acquired in different times and generates high-spatiotemporal resolution data by calculating the weight of different changes. This method provides an excellent fusion approach for spatiotemporal fusion because the results show high accuracy. However, the results would be poor when the type of land cover changes or the cover area is heterogeneous. Third, the learning-based method is based on the development of compressed sensing and sparse representation technology. This method represents a recent development that relies on learning the relationship and difference of multi-source satellite images by training samples and constructing an image dictionary. Although the learning-based method could obtain good results, the processing efficiency is lower than that of other methods, and it requires the training of sample selection.
Recently, the result of spatiotemporal fusion has been used in various applications, especially in the reconstruction-based method. This method is mainly used in time series data analysis as well as in retrieval and regional data set generation. For time series data analysis and retrieval, many researchers have used the results in developing the missing images of time series, detecting phenology, inversing urban environment parameters, estimating gross primary production, evaluating biomass, calculating land surface temperature, and so on. Given that the covered area of a low spatial resolution is large and the spectrum continuity of spatiotemporal fusion results is high, these results could be applied to the generation of regional data sets.
Although the spatiotemporal fusion method has seen considerable development, certain problems remain. The uncertainties are attributed to the complexity of land cover change, the errors of sensor calibration, and the data pretreatment process. The five potential aspects of the spatiotemporal fusion method that require further study are the consistency of data from different sensors, introduction of nonlinear mixed models, addition of prior knowledge, introduction of deep learning theory, and expansion into other satellites.