下载中心
优秀审稿专家
优秀论文
相关链接
摘要
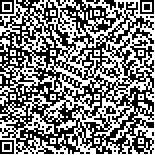
随着对地观测技术的发展,海量遥感影像不断传输到地面。传统的遥感信息处理方法在处理效率、精度上的不足,限制了遥感信息的挖掘及利用,亟需发展智能化方法满足遥感影像处理的需求。受自然界中生物进化机制的启发,基于进化计算的遥感影像智能化处理方法具有以下特点:(1)拥有全局优化能力,对目标函数的优化能力更强;(2)具有自组织、自学习的特点,能够从遥感数据本身学习,不依赖数据分布等先验信息;(3)拥有处理多目标问题的能力,同时考虑多个目标函数而不需要人工确定它们之间的权重。因此,智能化遥感信息处理方法能够在海量遥感影像中有效地提取适用于不同应用目的的信息。本文主要介绍智能化遥感信息处理方法的典型应用包括遥感影像智能化分类(监督分类、聚类)、遥感影像亚像素信息提取(高光谱影像混合像元分解、亚像元制图),并讨论了遥感信息智能化处理方法的发展方向。
With the development of earth observation techniques, a large number of high-resolution remote sensing images can now be acquired by using different types of sensors. Handling these "big" remote sensing data with diverse characteristics is difficult when traditional remote sensing techniques are used. New challenges, such as high-dimensional datasets (high spatial and hyperspectral features), complex structures (nonlinear and overlapped distribution), and optimization problems (high computational complexity), have also emerged. To address these problems, evolutionary computing-based techniques based on biological systems have been widely used for remote sensing image processing. Such techniques possess the following advantages:(1) powerful global optimization capability, acquiring the optimal or nearly optimal solution of objective functions; (2) self-organizing and self-learning capability, learning from original remote sensing data autonomously; and (3) capability of handling multi-objective problem, optimizing the multiple objective function simultaneously because of its population-based characteristics. Evolutionary computing has achieved preliminary success in the field of remote sensing data processing. In this paper, the applications of evolutionary computing to the fields of remote sensing image processing are reviewed, along with feature representation and feature selection, classification and clustering, and sub-pixel-level processing techniques, such as endmember extraction, hyperspectral unmixing, and sub-pixel mapping. Compared with the traditional methods of remote sensing image processing, these new methods are thought to be intelligent and accurate because of their powerful global optimization. For example, their constraints on the characteristics of objective functions, such as their derivatives, are few. They can also generate few assumptions about remote sensing data because of their self-organizing and self-learning capabilities. They can consider a large number of objective functions because of their capability of handling multi-objective problems. In summary, these new methods exhibit intelligent characteristics and high accuracy in remote sensing image processing. Finally, several crucial issues and research directions in the use of evolutionary computing are highlighted:(1) multi-objective optimization for regularization-based ill-posed problems in remote sensing processing, such as hyperspectral unmixing; (2) the discussion on the efficiency of evolutionary computing-based remote sensing processing methods, such as the memetic algorithm, which is a hybrid of evolutionary computing and machine learning, and the speeding up techniques of evolutionary computing-based remote sensing processing methods.