下载中心
优秀审稿专家
优秀论文
相关链接
摘要
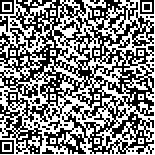
遥感数据反演高时空分辨率NDVI对监测植被动态变化过程具有重要意义,然而受天气影响,单颗卫星难以提供时间连续的高空间分辨率NDVI数据。以华北平原中东部为实验区,联合HJ-1 CCD数据和MODIS数据,对STARFM算法进行了改进,(1)考虑了不同地物对光谱响应的差异,为减少分类错误利用统计学上(x±2σ)对分类数据进行筛选,按照不同地物类型分别利用线性拟合方法修改光谱距离权重;(2)定义了预测半径,对HJ-1 CCD数据因外界影响而缺失的影像进行了预测。结果表明,与真实影像相比,预测结果呈现了较好的空间一致性,相关系数均达到了极显著相关,改进算法的预测精度要高于原算法。利用该方法将HJ-1 CCDNDVI的空间变化信息与MODIS NDVI时间变化信息有机结合重构了高时空分辨率NDVI序列,有效补充了HJ-1CCD NDVI的缺失数据集。
Retrieval of Normalized Difference Vegetation Index (NDVI)with high spatial and temporal resolutions from remote-sensing data is important for monitoring the dynamic changes in vegetation. However, owing to the impact of weather, a single satellite cannot provide a time series with high-spatial-resolution NDVI data. An effective solution to this problem is to fuse the NDVI with various resolutions retrieved from different sensor data to produce a dataset that has both high spatial and high temporal resolutions. In this study, the middle east part of North China Plain is selected as the experiment area, and HJ-1 CCD and MODIS NDVI data are used. The spatial and temporal adaptive reflectance fusion model is improved in two ways:(1)The difference of the spectral responses between different objects is considered using the confidence interval (x±2σ) to filter the data and consequently reduce classification errors, and the linear regression method is used to modify the spectral distance weight according to different objects. (2) The predicted radius is defined to predict the missing HJ-1 satellite data whose unavailability is caused by external influences.
Results indicate that the distribution of high and low NDVI values of the six studied dates is highly consistent between the observed and predicted images. According to the results of the correlation analysis, the scatter points concentrate along the line of y=x. The correlation coefficients of the six pairs of observed and predicted NDVI images are 0.957, 0.962, 0.935, 0.964, 0.913, and 0.933, which are all significant at 0.01 levels, and all RMSEs are less than 0.07, which indicates good fusion results. According to the difference images and their histograms, the averages of the six difference images are -0.02, 0.001, -0.012, -0.025, 0.023, and -0.02, with standard deviations of 0.049, 0.044, 0.049, 0.036, 0.056 and 0.043, respectively. The pixel values concentrate around 0, and 90% of the pixels have absolute values smaller than 0.1. The percentages of pixels that have absolute values smaller than 0.2 are 99.80%, 99.97%, 99.95%, 99.98%, 99.79%, and 100% for the six images. The accuracy of results tends to decrease as the time intervals increase between the base and prediction dates. The comparison of the results of IR shows that the prediction accuracy of the improved algorithm is higher than that of the original algorithm. The results also show that the base and predicted images have similar seasonal characteristic. If the time interval is extremely long, however, similar seasonal characteristics are not obvious or exhibit significant differences, which reduce the accuracy and precision of the prediction results. Therefore, an appropriate reference image should be selected.
In summary, the improved method is employed to reconstruct NDVIs with high temporal and high spatial resolutions by combining the spatial details of HJ-1 CCD data and the temporal variation of MODIS data to effectively supplement the missing HJ-1 CCD NDVI dataset.