下载中心
优秀审稿专家
优秀论文
相关链接
摘要
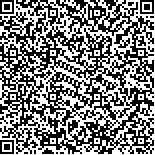
从低层视觉特征与地物空间关系特征对影像内容进行描述,建立检索模板与目标影像间的相似性直方图表达,提出一种适用于高分辨率遥感影像检索的新方法。首先,利用Quin+树将大幅面原始遥感影像分解为一系列同尺寸的序列子块;然后,分别提取各子块的低层视觉特征与地物关系特征,并以子块为基元构建候选子块的特征直方图;最后,对比检索模板与候选子块间的特征直方图相似性,实现高分辨率遥感影像的检索。使用多幅多源高分辨率遥感影像进行实验,结果表明本文方法对耕地、水系、建筑物等地类的检索精度大都维持在0.8以上,且各项检索性能指标均优于已有的两种遥感图像检索算法。
Image retrieval is a key technology for data acquisition and knowledge transformation under the background of remote sensing data. Remote sensing images with high spatial resolution provide Object details and diverse structures of land features, thereby making differences in local visual feature as evident. Most existing retrieval methods represent and model image contents on the basis of low-level visual features of the image, leading to limited retrieval performance of high-resolution remote sensing image. This paper presents a novel retrieval method for high-spatial resolution remote sensing image; the proposed method utilizes abundant information about the spatial distribution and structure of land features.
In the proposed method, data on low-level visual features and land-feature relations are utilized to represent the content of remote sensing images. Firstly, Quin+ tree is used to decompose the original large-sized image into a feature block sequence with a fixed size. Lowlevel visual features and land-feature relation descriptions are then extracted from the corresponding feature block. Feature histograms for candidate blocks are constructed according to the descriptions of the feature blocks. In each feature block, low-level visual information is represented using color and texture histograms. Moreover, land-feature spatial relation information is modeled as Object-direction and category co-occurrence histograms. Finally, the similarity between the query template and all candidate blocks is determined according to the feature histograms. Candidate blocks with high similarity values are selected as the final retrieval results.
Several high-resolution remote sensing images of QuickBird and ZY-3 are used in the experiments to confirm the effectiveness of the proposed method. Based on the retrieval results of the proposed method, the average retrieval precision of water, farmland, buildings, and other categories are higher than 0.75. In addition, the proposed method is compared with two typical CBIR methods. Quantitative evaluation indicates that the proposed method yields optimal results. The proposed method can significantly improve the retrieval performance because it considers the description of space relationship information among different land features.