下载中心
优秀审稿专家
优秀论文
相关链接
摘要
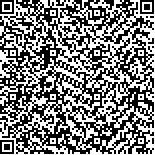
利用模拟数据,评价Autonomous Atmospheric Compensation (AAC)算法的抗噪性,认为AAC算法的抗噪性较弱。基于TASI实测数据,利用AAC算法开展反演计算时,计算结果呈现出多样性问题。结合In-sceneAtmospheric Compensation (ISAC)算法中黑体像元的标定方法,提出了一种复合改进算法。首先,利用ISAC算法反演的大气透过率和路径辐射,重新计算AAC算法中大气透过率之比(Tr)和相邻两强弱吸收通道的路径辐射之差(Pd),再次,运用经验公式获得稳定的大气反演结果(大气透过率和路径辐射),有效解决了计算结果多样性的问题。利用复合改进算法,开展的温度与发射率分离实验,证明反演得到的发射率波谱更接近野外实测波谱。
Land surface temperature and emissivity are essential parameters for quantitative thermal infrared remote sensing. These parameters can be accurately determined by converting at-sensor radiance into at-surface radiance through removal of effects related to atmospheric radiation and absorption. Therefore, obtaining accurate atmospheric parameters is the fundamental of quantitative thermal infrared remote sensing. Atmospheric parameters are typically obtained using a hyperspectral image through (1) Autonomous Atmospheric Compensation (AAC) and (2)In-Scene Atmospheric Compensation (ISAC) algorithms. Through simulation studies, we found that the noise immunity of the AAC algorithm was weak. When this algorithm is applied to images derived from Thermal Airborne hyperspectral Imager (TASI), results of atmospheric inversion calculation are uncertain. However, the ISAC algorithm can select blackbodies by linear regression, thereby resolving "AAC uncertainty." Based on the combination of these two algorithms, an improved algorithm is proposed to obtain accurate atmospheric parameters for TASI data analysis. Pixel brightness temperature of each TASI data channel was calculated, and the channel with the highest temperature was selected. The ratio of the number of pixels with the highest brightness temperature to the total number of pixels in the channel was then determined, and the channel with the highest ratio was used as reference channel. The maximum pixels of this channel were selected as test pixels to determine surface temperature. The obtained surface temperature and Planck function were used to build a linear regression model for extracting atmospheric transmittance and path radiance data based on the Kolmogorov-Smirnov statistic. The model was used to easily obtain transmittance radio (Tr) and path radiance difference between strong and weak absorption channels (Pd).MODTRAN was then applied to simulate atmospheric spectra based on the condition of the study area. The simulated pseudoTASI32-band spectra were resampled. Basing on the resampled atmospheric spectra, we determined empirical formula coefficients, goodness of fit, and root mean square error. The empirical formula was employed to obtain atmospheric transmittance and path radiance. The diversity of the two parameters(Tr and Pd) is effectively controlled using the proposed algorithm. The inversion results are more stable than that obtained through the AAC algorithm, particularly at the spectral position of absorption peaks. Moreover, compared with the retrieved data from the improved algorithm, the simulated results by MODTRAN are less reliable because they could not ascertain differences in time and space. We applied the inversion result of the improved algorithm and the simulated result of MODTRAN to experiments on Temperature and Emissivity Separation (TES) by using the ASTER-TES algorithm to determine the emissivity spectra. The emissivity spectrum recovered from the improved algorithm is more similar to the spectrum measured in field by using MORDTRAN. The improved algorithm, similar to the ISAC algorithm, can select blackbodies by linear regression to restrain the diversity of Tr and Pd in the AAC algorithm. Despite the accuracy of the retrieved atmospheric spectra or the recovered emissivity spectra, the improved algorithm performs better than the AAC algorithm and MODTRAN when applied to TASI data. Future studies must investigate whether the improved algorithm can be applied to other types of data.