下载中心
优秀审稿专家
优秀论文
相关链接
摘要
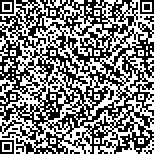
高空间、时间分辨率遥感数据在监测地表快速变化方面具有重要的作用。然而,对于特定传感器获取的遥感影像在空间分辨率和时间分辨率上存在不可调和的矛盾,遥感数据时空融合技术是解决这一矛盾的有效方法。本文利用像元分解降尺方法(Downscaling mixed pixel)和STARFM模型(Spatial and Temporal Adaptive Reflectance Fusion Model)相结合的CDSTARFM算法(Combination of Downscaling Mixed Pixel Algorithm and Spatial and Temporal Adaptive Reflectance Fusion Model)进行遥感数据融合。首先,利用像元分解降尺度方法对参与融合的MODIS数据进行分解降尺度处理;其次,利用分解降尺度的MODIS数据替代STARFM模型中直接重采样的MODIS数据进行数据融合;最后以Landsat 8和MODIS遥感影像数据对该方法进行了实验。结果表明:(1) CDSTARFM算法比STARFM和像元分解降尺度算法具有更高的融合精度;(2)CDSTARFM能够在较小的窗口下获得更高的融合精度,在相同的窗口下其融合精度也高于STARFM;(3)CDSTARFM融合的影像更接近真实影像,消除了像元分解降尺度影像中的"图斑"和STARFM模型融合影像中的"MODIS像元边界"。
High spatial and temporal resolution remote sensing data play an important role in monitoring the rapidly changing information regarding the earth's surface. However, the spatial and temporal resolutions of a remote sensing image for a specific sensor contain irreconcilable conflict. Fusing the spatial and temporal features of different remote sensing images to generate high-spatial-temporal remote sensing data is an effective method to solve the contradiction. This paper aims to improve the fusion data performance of STARFM in heterogeneous areas by downscaling rather than directly resampling a coarse pixel.The combination of the downscaling mixed pixel method and the spatial and temporal adaptive reflectance fusion model (STARFM) (CDSTARFM) was proposed. In this approach, the downscaling mixed pixel method first reduces the MODIS data that participated in the fusion. Then, the downscaled MODIS data replaces the direct resample MODIS data that appeared in the original STARFM. Finally, the subsequent steps of the STARFM are completed to predict Landsat-like images. The proposed algorithm produced high-resolution temporal synthetic Landsat 8 data based on Landsat 8 and MODIS remote sensing images.Results show that the CDSTARFM was more accurate than STARFM and downscaling methods. The downscaled data used in the CDSTARFM can more fairly reflect surface information than the resampled data of MODIS, which increase the probability of "pure pixels" and allow the CDSTARFM method to more easily determine the "pure" similar pixels in the search window. Therefore, the three indicators[i.e., correlation coefficient (r), root mean square error (RMSE), and the Erreur Relative Globale Adimensionalle de Synthèse (ERGAS)] as well as the scatterplots and visual effect of synthetic images for the CDSTARFM are better than those obtained by STARFM and downscaling methods. Moreover, the optimal window size (11×11 OLI pixels) of the CDSTARFM is smaller than that of the STARFM (31×31 OLI pixels). The accuracy of the CDSATRFM at the same window size is higher than that of the STARFM. The predicted NIR band is evaluated as an example in this study. The correlation coefficients (r) for the CDSTARFM, STARFM, and downscaling methods were 0.96, 0.95, 0.90, respectively; RMSEs were 0.0245, 0.0300, 0.0401, respectively; and ERGAS were 0.5416, 0.6507, 0.8737, respectively. Moreover, synthetic images effectively eliminated the "homogeneous spot" that appeared in the fusion images predicted by the downscaling algorithm and the "boundary of MODIS pixel" that appeared in the synthetic images produced by the STARFM.The temporal-spatial fusion of remote sensing data is an effective approach to solve the conflict of temporal and spatial resolutions of a sensor. This paper used the CDSTARFM algorithm, which combines downscaling method and STARFM to fuse remote sensing data. The CDSTARFM algorithm was verified by the experimental data of Landsat 8 and MODIS images, and it was compared with the STARFM and downscaling methods. The conclusions are listed bellow:(1) CDSTARFM using the downscaled data presented improved results to replace the directly resampled data used in STARFM. The three indicators (i.e., r, RMSE, and ERGAS) and the scatterplots for the CDSTARFM are the best compared with those for STARFM and downscaling methods. (2) The window size at which the best synthetic images were predicted by CDSTARFM is smaller than that by STARFM. In addition, CDSTARFM exhibits better accuracy than STARFM and downscaling methods at the same window size. (3) The synthetic images produced by CDSTARFM are more visually similar to the reference images, especially in the fragmented regions. CDSTARFM can eliminate the homogeneous "plot" in the synthetic images that were generated by downscaling methods and the "MODIS pixel boundary" in the prediction images generated by STARFM in fragmented landscapes.