下载中心
优秀审稿专家
优秀论文
相关链接
摘要
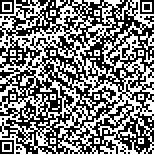
矿物的混合多属于致密型混合,在可见光-短波红外波段的混合呈现非线性特征,同时由于矿物混合的复杂性以及图像中完全纯净的像元可能不存在等原因,使得从图像上提取端元具有较大不确定性。本文根据矿物单次散射反照率的线性可加性,提出一种基于矿物单次散射反照率光谱库的稀疏解混算法,利用Hapke模型将矿物反射率转换成矿物单次散射反照率,构建矿物单次散射反照率光谱库,以半监督的方式通过稀疏回归的方法从光谱库中寻找最优端元组合,并估算混合像元中各端元的丰度。利用RELAB矿物混合光谱库进行算法验证,结果表明,丰度反演的平均绝对误差为3.12%;将本文方法应用于美国内华达州铜矿区的AVIRIS高光谱图像数据,所得丰度图与美国地质勘探局USGS矿物识别结果具有较好的一致性。本文算法不需要从图像提取端元,并且考虑到了矿物的非线性混合特征,能够得到较高的反演精度,在近地行星和卫星表面岩矿成分的探测等领域具有较好的应用前景。
Mineral mixtures are intimate mixtures have been extensively generated both on Earth and on other planets. The scatter of the particles that comprise mineral mixtures typically initiates complex optical interactions that are not interpreted by a linear mixing model. Moreover, the extraction of endmember signatures from the original data may be difficult given complex mineral mixtures and the lack of completely pure spectral signatures in the scene. Thus, we propose a sparse unmixing algorithm based on the spectral library of a Single Scattering Albedo (SSA) to decompose intimate mixtures.The sparse unmixing method aims to determine the optimal subset of endmembers from a spectral library and to estimate its fractional abundances in each pixel. The data from the spectral library are considered prior information; thus, sparse unmixing does not rely on endmember extraction algorithms. The Hapke model can describe intimate mixing effects effectively, and SSA can be obtained based on the theory behind this model. The SSA of the component particles is a linear mixture; therefore, linear unmixing techniques can be applied in the SSA space instead of in the reflectance space. The data processing procedure in this study mainly consists of three steps:(1) Building the spectral library of reflectance and then resampling the library spectrum to wavelength range and position; (2) Converting the reflectance to SSA and constructing a SSA spectral library; (3) Sparse unmixing.The laboratory spectra of the mineral mixtures and of the AVIRIS Cuprite data set are used to verify our method. We can identify the endmembers of mixtures accurately given laboratory data, and the mean absolute retrieval error of abundance is 3.12%. The qualitative analysis of AVIRIS hyperspectral data indicates that the abundance maps derived with our method are consistent with the Tricorder maps of United States Geological Survey (USGS), particularly in places where abundance is high; nonetheless, the abundance maps determined with our method still do not confirm to Tricorder maps to some extent. The classification maps of hyperspectral data consider each pixel to be pure, and each pixel is classified as the class of the representative endmember in the pixel. By contrast, unmixing classifies the scene at subpixel level, and the abundances represent the proportion of endmembers in a pixel. Experiment results show that our method can identify endmembers from the spectral library accurately, and can also estimate abundance well. The Hapke model can simply be used to minimize errors by calculating SSA directly. In our future work, we will calculate SSA exactly based on physical mineral parameters.