下载中心
优秀审稿专家
优秀论文
相关链接
摘要
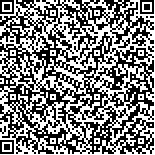
随着模式识别、机器学习、遥感技术等相关学科领域的发展,高光谱遥感影像分类研究取得快速进展。本文系统总结和评述了当前高光谱遥感影像分类的相关研究进展,在总结分类策略的基础上,重点从以核方法为代表的新型分类器设计、特征挖掘、空间-光谱分类、基于主动学习和半监督学习的分类、基于稀疏表达的分类、多分类器集成六个方面对高光谱影像像素级分类最新研究进行了综述。针对今后的研究方向,指出高光谱遥感影像分类一方面要适应大数据、智能化高光谱对地观测的发展前沿,继续引入机器学习领域的新理论、新方法,综合利用多源遥感数据、多维特征空间互补的优势,提高分类精度、分类器泛化能力和自动化程度;另一方面要关注高光谱遥感应用的需求,突出高光谱遥感记录精细光谱特征的优势,针对应用需求发展有效的分类方法。
Studies on hyperspectral remote sensing image classification have developed rapidly with the progress of related disciplines, including pattern recognition, machine learning, and remote sensing technology. This review generates a systematic summary and conducts a comprehensive evaluation of the advancements in current techniques for hyperspectral remote sensing image classification. Based on an overview of different classification schemes, we examine the recent progress in per-pixel classification algorithms for hyperspectral images from six aspects, namely, new classifier design(e.g., kernel-based methods), feature mining, spectral spatial classification, active and semi-supervised learning, sparse representation for classification, and multiple classifier systems. Future research directions are discussed as well. On the one hand, new theories and methods of machine learning should be introduced continuously into hyperspectral image classification. Moreover, multisource data and multidimensional feature spaces may improve the accuracy, generalization capability, and automation degree of a classifier. On the other hand, new classification methods should be designed in consideration of practical requirements to meet the needs of real applications and to emphasize the advantages of fine spectra in hyperspectral remote sensing.