下载中心
优秀审稿专家
优秀论文
相关链接
摘要
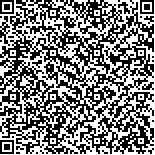
目前普遍采用的分类器通常都是针对单一或小量任务而设计的,在小数据量的处理中能取得比较满意的结果。但对于海量遥感数据的处理,其在处理时效和分类精度方面还有待研究。本文以遥感图像场景分类任务为例,着重对遥感数据分类问题中几种典型分类方法的适用性进行比较研究,包括K近邻(KNN)、随机森林(RF),支持向量机(SVM)和稀疏表达分类器(SRC)等。分别从参数敏感性,训练样本数据量,待分类样本数据量和样本特征维数对分类器性能的影响等几个方面进行比较分析。实验结果表明:(1)KNN,RF和L0-SRC方法相比RBF-SVM,Linear-SVM和L1-SRC,受参数影响的程度更弱;(2)待分类样本固定的情况下,随着训练样本数目的增加,SRC类型分类方法的分类性能最佳,SVM类型方法次之,然后是RF和KNN,在总体分类时间上呈现出L0-SRC >L1-SRC >RF >RBF-SVM/Linear-SVM >KNN/L0-SRC-Batch的趋势;(3)训练样本固定的情况下,所有分类方法的分类精度几乎都不受待分类样本数目变化的影响,RBF-SVM方法性能最佳,其次是L1-SRC,然后是Linear-SVM,最后是RF和L0-SRC/L0-SRC-Batch,在总体分类时间上,L1-SRC和L0-SRC相比其他分类方法最为耗时;(4)样本特征维数的变化不仅影响分类器的运行效率,同时也影响其分类精度,其中SRC和KNN分类器器无需较高的特征维数即可获得较好的分类结果,SVM对高维特征具有较强的包容性和学习能力,RF分类器对特征维数增加则表现得并不敏感,特征维数的增加并不能对其分类精度的提升带来更多的贡献。总的来说,在大数据量的遥感数据分类任务中,现有分类方法具有良好的适用性,但是对于分类器的选择应当基于各自的特点和优势,结合实际应用的特点进行权衡和选择,选择参数敏感性较小,分类总体时间消耗低但分类精度相对较高的分类方法。
The classification of remote sensing data plays an important role in all stages of remote sensing data processing and analysis. With the increase in the volume of remote sensing data, new problems concerning remote sensing big data classification tasks arise. Currently, the commonly used classifiers are usually designed for simple tasks to provide satisfactory results. However, for the processing of large volumes of remote sensing data, the scalability of classification efficiency and precision should be further investigated. Therefore, this study emphasizes on the comparisons of the scalability of typical remote sensing data classification methods to achieve this goal. Method:This study takes remote sensing image scene classification as an example and selects four well-known classification methods for comparison, namely, K Nearest Neighbor(KNN), Random Forest(RF), Support Vector Machine(SVM), and Sparse Representation-based Classifier(SRC), to conduct scalability analysis. The comparisons are conducted in terms of parameter sensitivity, effect of training sample data volume on classifier performance, effect of testing sample data volume on classifier performance, and effect of feature dimension on classifier performance. Results:The experimental results are as below:(1) The classifiers of KNN, RF, and L0-SRC are less parameter-sensitive than the classifiers of RBF-SVM, Linear-SVM, and L1-SRC.(2) In cases where the samples to be classified are fixed, all the classifiers tend to increase with the increase in the number of training samples. The SRC-type classification methods have the highest accuracy, followed by the SVM-type classification methods, the RF, and the KNN classifiers. In terms of overall classification time, the results show that the methods can be ranked as below:L0-SRC >L1-SRC >RF >RBF-SVM/Linear-SVM > KNN/L0-SRC-Batch.(3) In cases where the training samples are fixed, the classification accuracies of all the classifiers are seldom affected by the number of samples to be classified, which may be due to the learning abilities of all the different classifiers.(4) The feature dimension affects the efficiency and accuracy of different classifiers, in which SRC and KNN can obtain satisfactory results without high feature dimensions. SVM is tolerant to high feature dimensions and has a good learning ability with such high feature dimensions. By contrast, RF is insensitive to the increase in feature dimensions, and higher feature dimensions do not contribute much to the improvement of classification performance. Under such circumstances, the RBF-SVM exhibits the best performance, followed by the L1-SRC classifier, the Linear-SVM classifier, and the RF and L0-SRC/L0-SRC-Batch classifiers. In terms of overall classification time, the classifiers of L1-SRC and L0-SRC are the most time-consuming, whereas the other classifiers have relatively higher efficiency. Conclusion:Different classification methods have different advantages and disadvantages. In the tasks of classifying a large volume of remote sensing data, the selection of classifiers should be balanced and based on their characteristics and practical applications. Generally, a classifier that is less parameter-sensitive and less time-consuming during classification and obtains more accurate classification results is preferable.