下载中心
优秀审稿专家
优秀论文
相关链接
摘要
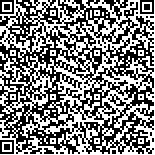
结合支持向量机SVM和马尔可夫随机场MRF在图像分类领域的优点,提出MRF-ν-SVC分类系统.与以往针对像素的分类不同,本系统在区域水平实现分类.首先通过双阈值准则判别区域间边缘的强弱,然后针对模糊边缘区域将基于MRF的空间语境模型改进为边缘语境模型,修正SVM原始问题中的偏差因子,从而优化最优分类超平面.实验表明,本文算法可有效提高分类精度,增强对斑点噪声的抑制能力,更好地分类特征相似的区域.
In this study, we put forward an MRF-ν-SVM classification system that integrates spatial contextual information with SVM while taking advantage of the good classification performance of SVM for small samples and its effective capacity to model the spatial correlation of MRFs. The system performs classification at the regional level instead of at the pixel level. To protect the image edge structure, we set up a dual threshold for local edge intensity and refine the MRF-based spatial contextual model to a contextual model for fuzzy edges. The bias of the original problems of SVM is then optimized to improve the optimal hyperplane.Sea ice is an important component of the global climate system. As a unique coherent imaging system, the Synthetic Aperture Radar (SAR) provides high-resolution images and serves as an important tool for monitoring sea ice. Sea ice image classification via SAR is thus an effective method for monitoring sea ice. This study integrates the spatial correlation based on MRF into the support vector classifier. Using the spatial contextual information based on MRF can improve scene interpretation accuracy. Meanwhile, SVM has good generalization ability and efficient classification performance, as well as high robustness to the Hughes phenomenon. MRF is thus used to model space interaction in the SVM framework.The watershed segmentation algorithm is used to obtain a number of homogeneous areas and the edges of closed regions. The image is then classified at the regional level. We extract the gray tone and three GLCM texture statistics, namely, entropy, contrast, and correlation, as the regional feature vector of the samples. For the characteristics of the sea ice image obtained via SAR, we use a window of a certain size to collect training samples and to record the edge information of each window image. The spatial contextual model based on the neighborhood system is optimized to the contextual model of the edge, which is introduced to the bias of the SVM, to structure the contextual bias of the edge and then correct the optimal hyperplane. To improve classification accuracy, we set up a double threshold for the strength of the edge. The labels of the regions for the strong edge are reserved, the edge label for the weak edge is cleared, and the contextual information for the fuzzy edge is computed. When the spatial correlation of images is not considered, several speckle-like regions can be observed in the classification maps of the KNN and ν-SVC algorithms. The regional MRF classification demonstrates weak noise resistance, and the classification result of the post SVM-MRF algorithm cannot accurately identify the regions with similar features. Experiments show that the proposed algorithm can effectively improve classification accuracy, enhance speckle noise resistance, and accurately classify the regions with similar features but different labels.The MRF-ν-SVM classification system that integrates spatial contextual information with SVM can effectively classify SAR images. The proposed algorithm has more advantages than the other four algorithms tested in the study. Specifically, it can accurately classify SAR images, reduce interference from speckle noise, and recognize regions with similar features but different labels.