下载中心
优秀审稿专家
优秀论文
相关链接
摘要
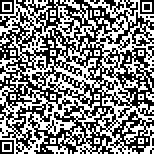
针对城市及周边区域建造区和自然地表交织分布的特点,探讨了利用归一化植被指数(NDVI)和归一化建造指数(NDBI)构造趋势面的地表温度(LST)降尺度方法,以北京市市区及周边较平坦区域为例实现了LST自960 m向120 m的降尺度转换。分析了LST空间分布特征及NDVI、NDBI对地物的指示性特征;以北京市四至六环为界分析NDVI、NDBI趋势面对地表温度的拟合程度及各自的适用区域;在120 m、240 m、480 m和960 m 4个尺度上评价了NDVI、NDBI和NDVI+NDBI趋势面对LST的拟合程度和趋势面转换函数的尺度效应;对NDVI、NDBI和NDVI NDBI等3种方法的降尺度结果分覆盖类型、分区域对比评价。实验结果表明结合两种光谱指数的NDVI NDBI方法降尺度转换精度有所改善,改善程度取决于地表覆盖类型组合。
Urban thermal environment studies require high spatial and temporal resolutions, while currently available remote sensors exhibit a compromise between spatial and temporal resolutions. Downscaling land surface temperature(LST)data with a low spatial resolution but high temporal resolution is a method to derive LST product at application-demanded spatial and temporal resolutions and has become a critical issue in thermal remote sensing applications. This study aims at downscaling LST from coarser spatial resolution(960 m)to finer(120 m)resolution by constructing a trend surface of spectral indices over highly heterogeneous urban areas, to lay a foundation for potential downscaling of MODIS LST to TM spatial resolutions. To describe the complex composition of built-up areas and vegetated surface in urban and surrounding areas, normalized difference built-up index(NDBI)and normalized difference vegetation index(NDVI)are combined to construct a trend surface to downscale LST from 960 m to 120 m. Urban areas in Beijing is taken as a study case. The key is to construct a trend surface of spectral indices to fit the LST, that is, to derive a trend surface transfer function at 960m, and then to use the transfer function established at 960 m to estimate LST at 120 m using spectral index trend surface at 120 m. To validate the applicability and stability of trend surface transfer function, the fitting degrees of spectral indices to LST are analyzed from the central urban areas to the sub-urban in Beijing and at varying scales from 120 m, 240 m, 480 m to 960 m; the scale effects of trend surface transfer function is also analyzed. The linear functions of transferring NDVI, NDBI and combined NDVI+NDBI to LST are established respectively to downscale LST from 960 m to 120 m. The results show that:(1)the LST in Beijing is the highest in urban central areas surrounded by the fifth ring road and is gradually decreasing from areas within fifth ring road to areas outside the sixth ring road; the built-up and bare soil areas have the highest average temperature values, while vegetation and water bodies have low average temperature values;(2)NDBI fitted the LST the best over the densely distributed built-up urban areas, while NDVI is more suitable to fit LST over naturally covered areas outside the sixth ring road; the combined use of NDVI NDBI can promote the fitting degrees to LST, depending on the composition of land cover types;(3)the correlation of spectral indices and LST applies on four varying scales from 120 m to 960 m. The errors induced by scale effects of transfer function are much lower than the errors brought by the models themselves;(4)the accuracy of downscaled LST using combined NDVI NDBI model is promoted, compared to those using a single spectral index. Yet the degree of accuracy enhancement depends on the composition of land cover types;(5)downscaling errors are related to aggregation errors. Although aggregation errors are higher than downscaling errors, they remain an important source of downscaling errors. The methods in this paper is also applicable to downscaling of MODIS LST data, while many factors such as temporal difference correction and geometric correction should be considered further.