下载中心
优秀审稿专家
优秀论文
相关链接
摘要
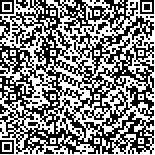
当前的遥感科学面临着遥感数据获取能力与数据应用能力之间突出的供需矛盾。尺度问题作为遥感科学中的关键问题,既限制了遥感作为一门科学向系统性、普适性的发展,又限制了遥感应用能力的发展。本文对定量遥感中的尺度问题进行了梳理,包括:遥感与传统站点观测之间的不一致、不同尺度遥感产品之间的不一致、机理模型的尺度适用问题,以及遥感产品与用户需求时空尺度间的不一致。对遥感中的尺度转换方法展开了讨论,总结了尺度转换的关键问题在于原数据信息量不足时引入额外信息和保留关键信息两方面。提出了构造地理要素趋势面的基本构想,搭建了一个具有普适性的尺度转换方法框架。核心内容是充分利用地表环境要素时间、空间上的信息作为先验知识,通过关联遥感观测新信息和先验趋势面生成指定时空尺度的地表要素产品。
Contemporary remote sensing science is challenged by serious supply-demand contradiction between data acquisition and application capabilities. Scale issue is attributed as a major and essential problem retarding the development of remote sensing in both theories and applications. Due to heterogeneity and complexity of land surface, scale issue naturally arises as how remote sensing observations match phenomenon or process on earth surface or how multi-scale remote sensing observations maintain consistency. Therefore, this paper endeavors to build up a universal scaling methodology framework, which would hopefully help interpret and transfer remote sensing products between sensor-defined scales and user-demand scales.
To begin with, scale issues in remote sensing are analyzed as: inconsistency between pixel-based remote sensing observation and conventional point-based field measurement, inconsistency between remote sensing products at varied scales, scale adaptabilities of physically-based models and scale inconsistency between remote sensing product and user demand. It is pointed out that multi-scale remote sensing information is related to characteristics of land surface and simple averaging or classic image processing will result unexplainable data.
Second, scaling methods are explored in terms of up-scaling and down-scaling. Up-scaling involves scaling from point-based field observations to pixel-based remote sensing observations and scaling between remote sensing products at varied scales. For the former a general way is to introduce extra information while the latter always uses two approaches as clumped or distributed computation. Preferred up-scaling is supposed to reserve key information, but most current methods tend to derive more homogeneous product of low information quantity. Down-scaling refers to derive data with more detailed information. The insufficiency of information of the original image is unavoidable and it is mandatory to introduce extra information. The earlier downscaling methods largely rely on pre-defined assumptions or interpolation, while a recent trend is to use relevant geographic features as prior knowledge. The central issue in scaling is concluded as how to reserve key information or how to introduce extra information.
Third, borrowing ideas of prior knowledge from quantitative remote sensing retrieval and trend surface in geographic applications, the paper proposes the concept of geographic trend surface and establishes a universal scaling methodology. The core is:(1)to construct prior knowledge warehouse by integrating all necessary information in retrieval and scaling, including link model, spectral knowledge, geographic trend surface, recent temporal change records and process models;(2)to adjust the geographic trend surface in spatial and temporal frame under the support of prior knowledge warehouse. All kinds of geographic information, including temporally stable geographic features, temporal change records, field observations and process models, are used to derive the spatial patterns of the target feature, based on which the spatial representativeness of observation at each location can be identified;(3)to update prior trend surface to post trend surface through Bayes theorem, where prior trend surface is expressed as prior knowledge and new remote sensing observations are taken as new knowledge;(4)to derive the product of land surface parameter at demanded scales based on error analysis and then update the post trend surface into prior knowledge warehouse. As a whole, construction of geographic trend surface lays the foundation of the methodology, which aims at addressing problems of information insufficiency or key information reservation in scaling. The paper provides a conceptual scaling model, while many technical details need to be fulfilled further in practice.