下载中心
优秀审稿专家
优秀论文
相关链接
摘要
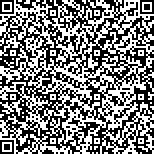
受海冰自身特性、成像系统特性和环境因素的影响,合成孔径雷达SAR海冰图像具有非平稳、尺度依赖的空间结构,现有的单马尔可夫随机场MRF模型分割方法只能较好地适应非平稳性,对海冰场景的多尺度结构考虑仍然是全局的.为此,本文提出了一种区域分裂过程与二叉树分层结构自适应更新相结合的单MRF图像分割方法.首先利用单MRF模型的全局迭代权值完成初始区域合并,同时以二叉树形式保护合并过程的记录.所设计的分层合并算法可保证二叉树结构的节点数与场景中的对象尺度具有正相关性.随后的细化分裂并不产生新的区域,只是返回到初始配置.依据场景中不同区域对象的尺度,自适应地调整空间语境模型中的尺度权值,实现区域更新.实验表明,该方法有效提高了带有多尺度结构SAR海冰场景的分割精度.
关键词:
海冰图像 区域分裂 二叉树 马尔可夫随机场MRF 空间语境模型Synthetic Aperture Radar (SAR) has become a tool for the effective and convenient monitoring of sea ice. It plays a significant role in both scientific research and business activities, such as ship navigation. Owing to the large volume of SAR sea ice data, the automated interpretation of SAR sea ice images is very important. Unfortunately, such interpretation involves numerous imaging characteristics and environmental factors. Hence, a strong change in the scattering coefficient of SAR sea ice can make sea ice images non-stationary. Under such condition, the segmentation of SAR sea ice images becomes challenging. Previous studies use the traditional method based on Markov Random Field (MRF), which can effectively improve the accuracy of SAR sea ice image segmentation. However, the improvements of the MRF method are only based on local edge strength. The scale dependence of sea ice scenes must still be considered. Owing to the non-stationary scale of complex SAR sea ice images, local weight must be immediately integrated into the adaptation of complex scenes in the segmentation algorithm. To improve the accuracy of complex SAR sea ice image segmentation, we propose a segmentation method with a hierarchical binary tree structure on the basis of region splitting and adaptive adjustment.The global iterative weights based on the MRF model are used to complete the initial region merging, and the merging process is described in the form of a binary tree. In the proposed hierarchical clustering algorithm, a positive correlation exists between the scale of the object in a scene and the number of binary tree nodes. The subsequent refinement of region splitting does not generate new regions but only reverts to a previous configuration. The scale weight of the spatial contextual model is adjusted adaptively according to the complexity of the objects in different regions in a scene. The updated weights renew the regional merging.The segmentation result obtained by the proposed algorithm is compared with that of three other algorithms, namely, C-MRF, V-MRF, and IRGS. The result of the C-MRF algorithm is too smooth and ignores many details. Meanwhile, the V-MRF algorithm cannot accurately identify large-scale regions. As for the IRGS algorithm, it is difficult to use when identifying regions with sea ice of varying complexities. The proposed algorithm considers both edge details and regional consistency. The overall accuracy and kappa coefficient of the proposed algorithm are higher than those of the other three algorithms. Experiment results show that the proposed method effectively improves the accuracy of SAR sea ice image segmentation, particularly for images of complex scenes.Compared with the existing single MRF method, the proposed method obtains better visual effects. Despite several studies on multi-scale random fields and continuous state modeling methods, the problem of modeling discrete fields with multi-scale structures remains unresolved. To address this gap, we use a single MRF to model a discrete field with spatial dependence and non-stationary structure. Such model can satisfy ice class business specifications by drawing on the natural ways of efficiently representing the spatial structure of different dimensions to avoid the low efficiency of several schemes.