下载中心
优秀审稿专家
优秀论文
相关链接
摘要
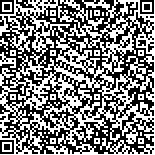
为了进一步提高基于独立分量分析ICA(Independent Component Analysis)的遥感图像变化检测精确度,更好地实现地表覆盖的动态监测,将多尺度几何分析和核独立分量分析KICA(Kernel Independent Component Analysis)相结合应用于遥感图像的地表覆盖变化检测.首先利用Contourlet变换、复 Contourlet变换CCT(Complex Contourlet Transform)、非下采样Contourlet变换NSCT(Nonsubsampled Contourlet Transform)等多尺度几何分析对土地遥感图像进行多尺度分解;然后对分解后的数据进行核独立分量分析,通过核函数将数据映射到高维特征空间中,再在该空间中用ICA方法分离出互相独立的分量;最后将分离后的地表变化分量转化为图像分量,再采用最大类间方差法对变化图像进行分割,实现地表覆盖的变化检测.给出了本文方法和近年来提出的基于ICA、基于KICA、基于小波变换和ICA等变化检测方法的实验结果,并进行了分析和定量比较.实验结果表明,基于多尺度几何分析和KICA的变化检测方法能更好地分离出遥感图像的变化信息,其中基于NSCT和KICA方法的错判和漏检误差最小,且在边缘细节方面处理得更好,而基于Contourlet变换和KICA方法的检测效率相对较高.
Remote sensing images are usually affected by light, angle, and other factors, thereby resulting in nonlinear mixed characteristics of a surface object spectrum. Thus, linear methods, such as Independent Component Analysis (ICA), have limitations. Kernel Independent Component Analysis (KICA) achieves nonlinear transformation through the kernel function, and the data are mapped into a high-dimensional feature space, where the data are analyzed by ICA. As a result, detection errors from nonlinear mixing of the surface object spectrum are considerably reduced. Remote sensing images are usually large and complex. If they are analyzed directly by KICA, the computation will be large. Therefore, we propose a change detection method of remote sensing images of land cover based on multi-scale geometric analysis and KICA. First, multi-scale decomposition of remote sensing images is conducted by using multi-scale geometric analysis methods, such as contourlet transform, complex contourlet transform, and Nonsubsampled Contourlet Transform (NSCT). The decomposed components are transformed into partitioned vectors, which consist of low-frequency and high-frequency components. The vectors are then analyzed by KICA and mapped into a high-dimensional feature space by the kernel function, so that the mixing pattern of vectors is linear. In the space, mutually independent components are separated by ICA. The change component of land cover is selected and transformed into an image component. The change image is transformed into a binary image by using automatic thresholding method, and the final change detection result is obtained.Experimental results of the proposed method and recently proposed methods based on ICA, KICA, as well as wavelet and ICA are presented. Analysis and quantitative comparisons are conducted. Based on subjective visual effects, the isolated points and discrete regions in the results obtained by the proposed method decreased compared with those obtained by the methods based on ICA, KICA, as well as wavelet transform and ICA. The land edge is fully retained in the case of less isolated points, thereby reflecting more accurately actual change information of the surface. According to objective quantitative indicators, such as erroneous error, omission error, overall accuracy, and running time, the proposed method is more accurate than the methods based on ICA, KICA, as well as wavelet transform and ICA. The overall accuracy of the method based on NSCT and KICA is the highest, whereas the method based on contourlet transform and KICA shows a relatively high computational efficiency.Our method can separate change information of remote sensing images better. The method based on NSCT and KICA exhibits the smallest misjudgment and misdetection errors and preserves edge details better. The method based on contourlet and KICA shows relatively high detection efficiency.