下载中心
优秀审稿专家
优秀论文
相关链接
摘要
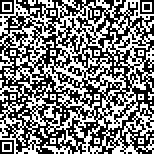
针对高分辨率卫星影像,提出一种特征分量构建与面向对象结合的阴影提取方法。分析遥感阴影光谱特性,构建彩色不变特征C3、亮度特征I、主成分第一特征量PC1以及蓝色波段和近红外波段归一化比率特征RATIOb_nir,增强阴影信息。采用线性变换将几个特征分量Digital Number (DN)值归一化到相同范围,对这几个分量进行综合分析。以I和PC1分量为输入对影像进行多尺度分割,建立包括波段均值、标准差、最大差异等特征的规则集,实现面向对象的阴影信息提取。选取20幅QuickBird影像为例进行阴影提取实验,平均总体精度为97%,平均用户精度为96%,平均Kappa系数为0.94。实验结果表明,相对传统基于像素信息提取方法,本文方法提取阴影斑块完整,无破碎图斑;相对基于原始光谱的面向对象方法,本文方法提取精度更高。
High-resolution satellite images provide extensive spectral, shape, and textural information of ground objects; as such, these images have been widely used in many fields. As by products of images, shadows affect the visual interpretation and automatic identification of landscape objects. Nevertheless, shadows reveal additional useful information, such as shape, height, surface characteristics, and relative position of targets. Therefore, studies should be conducted to develop methods for detecting shadows. To extract shadows accurately, researchers should consider the necessary pre-conditions; such methods should also be readily available for further utilization. This study proposed a shadow detection method based on object-oriented method and established characteristic components for high-resolution satellite images. To analyze the spectral characteristics of shadows, we determined several components, such as color invariant C3, brightness I, first principal component (PC1), and RATIOb_nir. We then used these components to highlight shadow areas in images. RATIOb_nir index, which can be used to distinguish shadow and water efficiently, was developed by considering the Rayleigh scattering of different wavelengths in shadow and non-shadow areas. However, such components are difficult to analyze comprehensively because different construction methods have revealed various value ranges. To overcome this problem, we used a linear normalization method and transformed the pixel values of images in the same range of 0 to 1. Object-oriented method, which comprised segmentation and information extraction, was used to extract shadow areas in the enhanced images. Brightness I and PC1, which contained relatively clear boundary information, were chosen as the main data source for multi-resolution segmentation based on the characteristics of high-resolution images. C3 and RATIOb_nir indices were also used the main data source in the subsequent classification. Several characteristics, such as mean value, maximum difference, standard deviation, area, and gray-level co-occurrence matrix, indicated the difference between shadow and non-shadow objects; as such, these characteristics were selected to extract shadow areas from images. Shadows in 20 QuickBird images were extracted using the proposed method and two contrast experiments. Data revealed that the average total accuracy of the proposed method was 97%, the average producer accuracy was 96%, and the average Kappa index was 0.94. The combined characteristic component-based and object-oriented methods could be used to obtain shadows with perfect shapes but without fragmentation compared with pixel-based method. The combined method also exhibited higher accuracy than the object-oriented method based on original optical images alone. Considerable experiments and statistically high-precision results of the proposed method showed that the combined characteristic component-based method and object-oriented method could be used efficiently to enhance the contrast of shadows against other features. Furthermore, this combined method could be used to ensure the complete extraction of shadow areas. The proposed method could be applied not only to QuickBird imagery used as test data in this study but also to other high-resolution satellite images.