下载中心
优秀审稿专家
优秀论文
相关链接
摘要
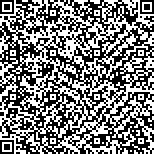
提出一种软硬变化检测的作物识别方法SHLUCD(Soft and Hard Land Use/Cover Change Detection Method)。该方法利用多期遥感影像能够有效表达作物的生长物候特征,以达到在离散变化区(即纯净像元区,包括完全转换成作物的突变区域和非作物区域)和连续变化区(即渐变区,混合像元区,是部分转化为作物的区域)准确进行作物的识别。在北京市选择一个研究区,以冬小麦为研究对象,选用2011年10月6日(播种期)和2012年4月16日(拔节期)两期环境减灾1号卫星影像,分别采用硬变化检测方法HLUCD(Hard Land Use/Cover Change Detection Method)、软变化检测方法SLUCD(Soft Land Use/Cover Change Detection Method)和SHLUCD进行冬小麦的识别。实验结果表明:在不同尺度窗口下,SHLUCD较传统方法表现出较明显的优势,具有更低的均方根误差RMSE(SHLUCD为[0.14,0.07],HLUCD为[0.15,0.07],SLUCD为[0.16,0.08])和偏差bias(SHLUCD为-0.0008,HLUCD为-0.007,SLUCD为0.014)和更高的决定系数R2(SHLUCD为[0.68,0.86],HLUCD为[0.62,0.86],SLUCD为[0.60,0.86])。针对冬小麦突变区域、冬小麦渐变区域和非冬小麦区域分别进行评价,表明SHLUCD识别精度接近各区最佳的识别方法,进一步验证了SHLUCD的灵活性和适用性。SHLUCD方法在离散变化区能够通过土地覆盖类型状态变化来有效地识别出冬小麦,在连续变化区可识别出土地覆盖的状态变化程度定量表达冬小麦的丰度,是其他作物多时相遥感变化检测的前期实验基础。
The Soft and Hard Land Use/Cover Change Detection (SHLUCD) was proposed to express the variation of crop phenology effectively, which will help identifying the target crop both in discretely and continuously changed regions (discrete region is in an area that was totally changed into crop and non-crop region; the continuously changed region is in a transitional area where part of the target crop is changed into crop). The method was applied in a sub-region of Beijing. Two HJ-1 CCD images that were utilized by Hard Land Use/Cover Change Detection (HLUCD), Soft Land Use/Cover Change Detection (SLUCD), and SHLUCD to extract winter wheat area in the study area; the images were acquired on October 6, 2011 (T1) and April 16, 2012 (T2) represent the period of sowing and jointing, respectively. The results showed that the crop distribution derived from the SHLUCD can get a higher accuracy than that from HLUCD and SLUCD. The crop distribution derived from the SHLUCD also performs better in scale evaluation than that derived from other methods; the former has lower RMSEs and bias and higher R2 value. The RMSEs of SHLUCD, HLUCD, and SLUCD are in the ranges of 0.14 to 0.07, 0.15 to 0.07, and 0.16 to 0.08, respectively; the biases of SHLUCD, HLUCD, and SLUCD are approximately -0.0008, -0.007, and 0.014, respectively; the R2 values of SHLUCD, HLUCD, and SLUCD are in the ranges of 0.68 to 0.86, 0.62 to 0.86, and 0.60 to 0.86, respectively. Accuracy assessment was also applied in abruptly changed area, continuously changed area and non-crop area respectively. It shows that the accuracy assessment results of SHLUCD are similar to that from the best method in all three regions. Therefore, our method is more flexible and suitable in crop mapping. The winter wheat mapping in discrete and continuously changed regions can be identified by the status of land use/cover change and land change scope through SHLUCD, respectively. Moreover, SHLUCD provides a new way for crop distribution mapping based on change detection.