下载中心
优秀审稿专家
优秀论文
相关链接
摘要
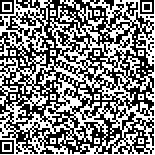
遥感数据的海量堆积与应用信息的匮乏日益凸显信息认知提取的重要性,在地学信息图谱方法论的指导下,同时参考视觉认知流程,提出了遥感信息图谱认知方法用于遥感数据的自动解译。在地理信息系统的统一框架下逐步挖掘多源遥感数据的“图”、“谱”特征并进行图谱耦合分析,通过多尺度分割、特征分析、监督学习等关键步骤完成“察觉-分辨-确认”的地学认知流程,初步满足自动化和智能化应用需求。在土地覆盖信息自动解译应用中建立了基于“图谱”先验知识的管理与运用机制以实现自动化,采用机器学习算法提升智能化程度,并以自适应迭代控制模型使结果精度向最优逼近。选取了珠江三角洲的试验区域进行了基于ALOS多光谱影像的土地覆盖自动分类,结果符合预期,说明了本文方法的可行性。
The importance of the extraction and cognition of geo-information has been increasingly highlighted in the face of the massive accumulation of remote sensing data and the lack of application information. According to the geo-informatics Tupu methodology regarding the visual cognitive process, Tupu-cognition can automatically interpret remotely sensed imagery. In this study, using a unified framework of geographic information systems, we extract the features of images step by step. Spatial-spectrum analysis is then executed in the geo-cognitive process described as "Perceive-Identify-Confirm". Algorithms like multiscale segmentation, feature analysis, and supervised learning are invoked to meet the application's requirements for automation and intelligence. In the cognitive application of land-cover information, we first establish the mechanism of prior knowledge management for automation. Second, a number of machine learning algorithms are employed to improve the intelligence. Finally, adaptive iteration is introduced to optimize the results. The data selected for this classification experiment are Advanced Land Observing Satellite (ALOS) multispectral images in the Pearl River Delta. The land cover results are consistent with expectations and illustrate the feasibility of our method.