下载中心
优秀审稿专家
优秀论文
相关链接
摘要
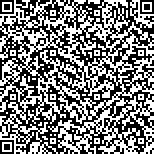
遥感估算叶面积指数(LAI)时空动态变化对全球气候变化研究具有重要的意义,为了提高遥感估算时间序列叶面积指数的精度,需要耦合遥感观测数据与LAI动态过程模型。本文提出一种基于双集合卡尔曼滤波(Dual EnKF)的时间序列LAI反演方法,同时更新LAI估计值和LAI动态过程模型中的敏感性参数,得到LAI和动态过程模型敏感参数的最优估计值来优化动态过程模型。一方面使得动态过程模型可以更好地描述LAI随时间的变化过程,降低模型预测误差,从而提高LAI动态过程模型的预测能力;另一方面通过耦合动态过程模型和辐射传输模型,集成遥感观测数据与动态过程模型的预测值,进而得到优化的LAI估计值。为检验算法,分别选取作物、草地和林地等典型植被验证站点进行Dual EnKF LAI时间序列估算,并分别与MODIS LAI产品及其SG滤波曲线、集合卡尔曼滤波方法反演LAI、未优化的动态过程模型模拟LAI结果进行比较,并配以一些站点地面实测点数据作为参考。结果表明,采用Dual EnKF方法得到的LAI不但保持了时间上的连续性,而且通过改善动态过程模型的预测能力,即使在缺乏高质量遥感观测数据时,也能够获得符合LAI发展趋势的估算值,没有出现跳跃、波动现象,时间序列曲线较稳定,更符合植被LAI变化规律,表明基于Dual EnKF的时间序列LAI遥感估算方法是提取LAI时间廓线的一种有效途径。
Leaf Area Index (LAI) is an important land surface parameter in global carbon cycle studies. Over the past decades, various of algorithms for retrieving LAI from satellite data have been developed. However, the continuity of LAI time series is still need to be improved. In this study, we present an algorithm for retrieving LAI dynamics based on the framework of Dual ensemble Kalman Filter (Dual EnKF), which updates the estimation of LAI and its sensitive parameters in a dynamic model, and gets optimal results simultaneously. The Dual EnKF algorithm can get better estimation results of the dynamic model, and assimilates remote sensing data into the dynamic model to get optimal estimation results of LAI. Three sites with the land cover of cropland, grassland, and forest are employed to validate this algorithm. The validation results show that the LAI temporal profile estimated by Dual EnKF method is very continuous with less fluctuations and abrupt change points; the estimation results of temporal dynamic model is improved, even when high quality remote sensing data is not available. LAI time series is in good agreement with the realistic LAI climatology.