下载中心
优秀审稿专家
优秀论文
相关链接
摘要
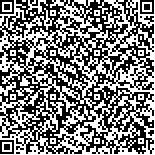
针对国产卫星数据特点及长条带影像匹配困难问题,提出了一种基于全球SRTM数据的影像匹配方法。本文探讨了长条带影像物理分块机制,并引入LBP/C算子实现了兴趣点的筛选。在全球SRTM数据的辅助下,采用投影轨迹法,建立了近似核线方程。沿核线方向,进行局部畸变改正,进而消除匹配窗口的几何变形与辐射差异,利用金字塔匹配策略,逐层进行相关匹配。最后,在原始层引入MPGC(Multi-photo Geometrically Constrained Matching)算法与RANSAC(Random Sample Consensus)算法,进行精化匹配,并剔除误匹配点。文中综合运用了小面元几何纠正法与基于控制网的匹配生长算法,从而提高了匹配点的精度与均匀性。本文方法可在并行环境下全自动实现不同分辨率、不同视角、不同时相的多轨道长条带影像匹配,获得高精度的同名点观测值。以天绘一号与资源三号卫星影像作为试验数据,与现有匹配算法进行对比结果表明该算法具有较好的鲁棒性,能够达到较高的匹配精度。
Faced with the problem of unstable reliability in matching long-strip imagery of Chinese satellite, a matching algorithm is presented using the global Shuttle Radar Topography Mission (SRTM) data as elevation control. First, this algorithm employs the block partition mechanism, and introduces Local Binary Pattern/Contrast (LBP/C) operator to filter the interest points. Second, the global SRTM data is used to compute the true topographic relief within the image coverage. Based on the true topographic relief, the approximate epipolar line is constructed and the accuracy is analyzed. Third, on the pyramid level, two-dimensional correlation matching is executed to search for the optimal matches along the epipolar line. During the matching process, the geometry rectification method is applied to improve the accuracy of matching. Finally, on the original level, Multi-Photo Geometrically Constrained (MPGC) matching algorithm is adopted to refine the matching result, and Random Sample Consensus (RANSAC) is imbedded to eliminate mismatches. In order to ensure the distribution uniformity of matches, the region-growing algorithm is introduced. The main advantage of the proposed algorithm is that it can realize the automatic matching for long-strip imagery of different Ground Sample Distance (GSD), different visual angles in parallel environment. Through the comparison between the proposed method and the mainly existing methods, the results show that the matching accuracy is improved.