下载中心
优秀审稿专家
优秀论文
相关链接
摘要
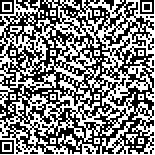
草地生物量的大区域精确估算对全球变化研究和草地资源的合理利用具有重要作用。为提高草地生物量的空间模拟精度,发展了草地地上生物量的高精度曲面建模方法HASM-GB(High Accuracy Surface Modeling for Grassland Biomass),基于内蒙古草地地上生物量野外调查资料和同时期的遥感影像数据,采用HASM-GB方法对内蒙古自治区草原地上生物量空间分布进行了模拟。通过野外实测数据采用修正的Jackknifing方法对HASM-GB方法模拟结果进行了验证,结果表明模拟结果与实测数据之间具有较低的均方根误差(28.03 g/m2)和较好的相关性,相关系数为0.62。将HASM-GB方法与遥感生物量回归模型、普通克里金和回归克里金3种方法进行了精度对比,结果表明与其他3种方法相比,HASM-GB方法的模拟结果具有相对较低的平均误差、平均绝对误差、均方根误差和与测定值较高的相关系数。通过对地上生物量空间分布格局模拟结果的分析可知,由于植被指数-生物量回归模型属于非空间方法,其模拟精度很大程度上取决于主、辅变量间的相关性水平,易受植被指数数据误差的影响。普通克里金不能考虑辅助变量的作用。HASM-GB方法能够充分考虑生物量采样点和辅助变量的空间变异信息和邻域样本的空间结构特征从而提高模拟精度,其对草地地上生物量的曲面模拟能力高于回归克里金。结果表明HASM-GB可以作为模拟草地地上生物量空间分布相对有效的方法。
The mapping of grassland biomass is of fundamental importance for estimating carbon budgets and the optimal use of grassland resources. The High Accuracy Surface Model for Grassland Biomass Simulation (HASM-GB) model was developed to estimate grassland aboveground biomass in Inner Mongolia, China. The ground truth biomass data and Normalized Difference Vegetation Index (NDVI) were used to predict maximum growing season biomass maps using the HASM-GB model. To evaluate the performance of HASM-GB, it was compared with three other methods: Satellite-Based Regression Model (REG), Ordinary Kriging (OK), and Regression Kriging (RK). As expected from theory, HASM-GB generally performs better than REG, OK, and RK, with a lower estimation bias, mean absolute error, root mean square error and higher correlation coefficient for measured and simulated values. From the predicted grassland biomass maps, the aspatial method vegetation index-biomass relationship technique was directly used with the variable NDVI to estimate biomass, and the precision of the results depend largely on how closely the primary and secondary variables are related. The spatial variation of the biomass produced by this method is very similar to the spatial variation of NDVI, so the simulation result is sensitive to errors in NDVI data. The OK method cannot factor information regarding vegetation index. However, HASM-GB can consider both the spatial structure of the measured biomass values and the NDVI data affecting local spatial trends, and it also had a higher precision of interpolation than RK. Consequently, HASM-GB is shown to be relatively effective for simulating spatial patterns of grassland biomass.