下载中心
优秀审稿专家
优秀论文
相关链接
摘要
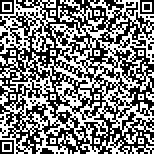
提出了一种基于Fisher权重分析的迭代光谱解混方法(WLSMA),该方法首先对高光谱图像进行区域分割,在分割后的各子块中自动提取端元;再次对提取的端元进行聚类,从光谱的整体特征上将不同类别的端元区分开,针对聚类结果中的每一类别各选取几个具有代表性的端元光谱,并对最优光谱进行窗口卷积处理,结合In_CoB指标构建端元光谱样本库;最后对图像进行迭代光谱解混处理,在丰度反演过程中引入基于Fisher准则的补偿权值矩阵以提高反演精度。AVIRIS高光谱数据实验证明,WLSMA不需要大量先验信息,利用Fisher准则和迭代光谱分析理论增强了相似性矿物的可分性,为加强对矿区地表岩性的认识和模拟提供了更大的灵活性和可能性,对高光谱矿物填图有一定的借鉴意义。
In the present study, an improved weighted iterative spectral mixture cycle called WLSMA is proposed based on the spectral unmixing method. First, this method divides the hyperspectral image into many regions and from each subregion endmembers are extracted automatically. Second, the extracted endmembers are clustered to distinguish different endmember classes from the overall type of spectra. We select the most representative endmember spectra from each class within the clustered results and process the optimal spectra using window convolution to establish an endmember spectrum sample library based on In-CoB. Third, an iterative spectral mixture cycle is applied to the image by introducing the compensation weighting matrix into the abundance estimation. The results of the AVIRIS data set indicate that WLSMA, which combines Fisher's principle and iterative spectral mixture theory, increases the separability between similar minerals without a large amount of prior information, and offers greater flexibility and the possibility to improve the understanding and modeling of real-world data.