下载中心
优秀审稿专家
优秀论文
相关链接
摘要
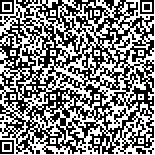
在归纳现有遥感地表温度降尺度方法的基础上, 选取3种代表性方法:Normalized Difference Vegetation Index (NDVI)、Pixel Block Intensity Modulation (PBIM)和Linear Spectral Mixture Model (LSMM)方法进行实验比较, 并建立了一种纹理相似性度量指标CO-RMSE (Co-Occurrence Root Mean Square Error)。结果表明:(1)NDVI方法受季节影响最严重, 不适于春、冬季, 其次为PBIM方法;(2)LSMM方法受分辨率限制最大, 低分辨率时丢失大量纹理信息, NDVI方法在较高分辨率时优于PBIM方法, 较低分辨率时则相反;(3)3种方法的适用区域分别为植被与裸土像元并存区域, 山区和反照率变化较大区域, 以及类别间温差较大区域;(4)NDVI方法操作最简单, LSMM方法最复杂。分析认为, 尺度因子是决定方法性能的关键, 应根据季节、分辨率、地表覆盖、应用目的和操作性等综合选择。
Remotely sensed Land Surface Temperatures (LSTs) usually have low spatial resolutions. Downscaling is an effective technique to enhance the spatial resolutions. Current methods for downscaling remotely sensed LSTs were summarized. Using satellite data, we made an inter-comparison among three typical methods, including the Normalized Difference Vegetation Index (NDVI) method, the Pixel Block Intensity Modulation (PBIM) method, and the Linear Spectral Mixture Model (LSMM) method. We further designed an index, Co-Occurrence Root Mean Square Error (CO-RMSE), for measuring the textural similarity in inter-comparisons. Results indicate that (1) the performance of the NDVI method is most affected by the season, followed by the PBIM method; (2) the performance of the LSMM method is most influenced by the spatial resolution; the NDVI method has an advantage over the PBIM method at high resolutions, while at low resolutions, the performance of the PBIM method is better than that of the NDVI method; (3) these three methods are suitable for areas with combination of vegetation and bare ground, areas with varied topography and albedo, and areas with distinct LST differences in different classes, respectively; (4) the NDVI method is the easiest to implement, while the LSMM method is the most difficult. Further analysis showed that scale factor is the key issue to the LST downscaling and it needs to be carefully selected regarding the season, spatial resolution, land cover, application and the operability.