下载中心
优秀审稿专家
优秀论文
相关链接
摘要
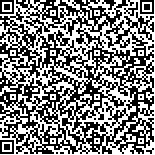
在喀斯特分布区,基岩、植被、裸地等多种地表覆盖交错分布,地物覆盖高度异质,并且呈现出短周期规律性变化和长期动态趋势变化,单一时相的影像进行土地覆盖分类精度非常有限.针对这一问题,本文提出一种顾及物候特征的多时相遥感影像分类策略,利用具有高时间分辨率的MODIS NDVI时间序列产品作为数据源,选择BFAST(Breaks For Additive Seasonal and Trend)方法进行NDVI时间序列的物候分解,采用动态阈值法对时序分解的物候轨迹进行标记,最后将物候标记特征与原始光谱时序综合特征进行组合,选择支持向量机(SVM)分类器进行土地利用覆盖分类,并且对比了不同特征空间下的分类结果.以云南省壮族苗族自治州丘北县和砚山县为研究区进行分类实验,结果表明,BFAST模型可以有效地分解出NDVI时序中的关键物候特征,相比基于单纯光谱特征的分类,物候驱动的喀斯特断陷盆地区土地覆盖分类精度有明显的提升,在NDVI、光谱和物候组合特征空间下,土地覆盖分类精度最高,总体精度和Kappa系数分别为88.94%和0.8693,尤其在灌木林、有林地、石旮旯地与稀疏植被的区分中,SOS、POS和GSG等物候特征具有较强的可分性,表明物候特征在地物识别中的有效性.
Karst regions are typical ecologically fragile zones constrained by a geological setting. In karst regions, vegetation, soil, and rock are cross-distributed or discontinuous, thereby resulting in high spatial and temporal heterogeneities of land cover. Land-cover classification based on a single optical image is generally not satisfactory. During the plant senescence period, for instance, the fractional cover of non-photosynthetic vegetation significantly influences the classification accuracy of bare land and sparse land. Meanwhile, the change in vegetation in karst regions is characterized by a combination of seasonal, gradual, and abrupt changes.
Concerning the aforementioned issue, this paper presents a novel phenology-driven land-cover classification strategy, which consists of performing Breaks For Additive Season and Trend (BFAST) for time-series decomposition, extracting phenological markers [e.g., start of season, end of season, length of season, base level of season, timing of mid-season, peak value of season, amplitude of season, rate of grow-up and senescence, gross spring greenness, and net spring greenness] from the phenological trajectory. Support Vector Machine (SVM) methods are also conducted to classify karst land cover. Specifically, a three-step strategy is proposed for phenology-driven land-cover classification. First, Normalized Difference Vegetation Index (NDVI) time series is derived from remotely sensed images acquired by Moderate Resolution Imaging Spectrometer (MODIS). BFAST approach is used to decompose the time series into seasonal, trend, and remainder components by integrating iterative change detection method. Second, with the decomposed seasonal and trend components of NDVI time series, seasonal parameters are extracted for the growing seasons. Finally, combined with the composited surface reflectance bands and vegetation index, phenological parameters are used to construct the decision space for SVM classifier.
An experiment is employed to validate the proposed method, in which the 4-year 16-day composite MODIS NDVI time series (2010—2013) located in Qiubei and Yanshan counties in Yunnan province, Eastern China is used for karst land-cover classification. Land-cover classification in different feature spaces of satellite image time series in 2013 is compared. Experimental results show that phenological information and the combination of both achieve better SVM classification performance than pure spectral information. The overall accuracy and kappa coefficient reach 88.94% and 0.8693 respectively. For the classification of shrub, sparse land, and upland, the improvement of their user accuracy and product accuracy is approximately 20%.
BFAST method can effectively decompose the NDVI time series of different land covers in karst regions into trend, seasonal, and remainder components. Differences among the phenological characteristics of land cover may improve their separability. Based on the extracted phenological markers from the phenological trajectory, land-cover classification in karst regions can be performed efficiently. However, according to the within-building classification decision space, the compositions of all phenological properties will bring in data redundancy, cost considerable time, and consume substantial computing resources. Efficient feature selection and optimization methods may be used to improve the classification performance of the proposed method. The spatial resolution of MODIS image is also a limiting factor.