下载中心
优秀审稿专家
优秀论文
相关链接
摘要
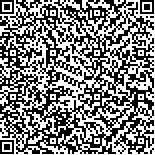
结合小波变换及字典学习提出了一种针对高光谱图像的压缩算法。该算法首先通过小波变换构建多尺度样本集,在小波域使用K-均值奇异值分解(K-SVD)方法学习得到原子尺寸不同的多尺度字典,然后在稀疏表示的过程中,定义一个原子使用频次筛选因子,通过统计局部最优波段稀疏表示时原子使用情况,结合筛选因子对字典原子进行优化筛选,使用精简后的字典对其余波段进行稀疏求解,最后针对不同尺度的表示系数采用自适应的量化编码。实验结果表明,与目前常用的3D-SPIHT和其他的多尺度字典学习算法相比,本文算法在中低比特率下,具有更好的重建性能。
Compression can significantly decrease hyper spectral images to relatively manageable sizes, thereby facilitating their efficient transmission and storage in aground station. Dictionary learning and sparse representation perform well in natural image compression. This study focuses on the properties of hyper spectral images and presents an efficient compression algorithm based on wavelets and dictionary learning for hyper spectral images.
First, multi-scale training samples are built through wavelet decomposition; the training samples of each scale are sent to the K-SVD dictionary learning model to obtainmulti-scale dictionaries by joint training, whichsimultaneouslycalculates the errors and update the multi-scale dictionary. Second, statistical analysis is performed on the used dictionary atoms in local optimal bands in the process of sparse coding, and a frequency selection factor is introduced. The statistical information and frequency selection factor are then used to decrease on-used or rarely used atoms in the dictionary. Other bands can be sparsely and easily represented using the simplified dictionary. Finally, the simplified dictionary is directly entropy coded, and the DC component is entropy coded after 4-neighborhood prediction and differential pulse code modulation. The indices of the coefficients of each scale are rearranged according to the numerical value and are separately entropy coded after DPCM. The sparse coefficients are also rearranged according to the sequential changing of indices and are entropy coded together after adaptive quantization. Results show that the proposed scheme outperforms the traditional spatial and other multi-scale dictionary learning algorithms. It is also much better than 3D-SPIHT in terms of bit rate distortion performance. As JPEG2000 (Part 2) largely benefits from the embedded block coding with optimized truncation strategy, it can achieve a better performance than our scheme. However, our proposed is much faster than JPEG2000 (Part 2).This study designed a novel hyper spectral image compressor based on wavelets and dictionary learning. Experimental results reveal that the proposed scheme outperforms the 3D-SPIHT and most compression algorithms. It is also much faster than the state-of-art compression standard JPEG2000 (Part 2). This compression algorithm can be improved further in the process of rearrangement before entropy coding. Our experiments prove that dictionary learning and sparse representation theories have great potential in hyper pectral image compression and interpretation applications. This study can motivate future research in this field.