下载中心
优秀审稿专家
优秀论文
相关链接
首页 > , Vol. , Issue () : -
摘要
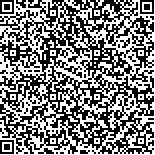
火星表面广泛分布的石块对于火星探测车的安全行驶构成潜在威胁,同时石块的分布特性也为研究火星着陆区域的地质演化提供了重要线索。然而,火星探测车图像中的石块识别面临多重挑战:石块和背景边缘模糊导致轮廓提取困难,表面纹理特征相似性引发误判,并且现有的火星石块真实数据集稀缺制约模型训练。为了实现火星探测车图像中石块的精确识别,本文提出一种基于卷积自注意力网络的石块自动识别模型,实现图像的像素级分割。该模型采取编码器-解码器架构,其中编码器基于卷积神经网络提取图像特征,并嵌入改进的自注意力模块以增强模型对上下文信息的感知能力;解码器则负责将编码器提取的特征映射回图像空间,实现精准分割。为了验证模型性能,本文对“祝融号”火星车影像进行标注,构建了天问数据集,结合模拟石块数据集Synmars、Simmars6k、“好奇号”影像数据集MarsData-v2等多个数据集对模型的性能进行测试和验证。此外,本文还将该模型与DeepLabv3+、Unet++、Segformer、Marsnet等多种方法进行精度比较。采用平均像素精度、召回率、交并比等指标进行评价,结果表明该模型能够准确识别石块,其在模拟数据集上精度和召回率均达到90%以上,在真实数据集上的精度和召回率均最优。
The Martian surface is characterized by a significant presence of rocks, whose size distribution, spatial density, and morphological characteristics are critical factors in determining the safety of landing site selection for Mars missions. These factors also directly influence the path planning and motion control of rovers during exploration activities. Moreover, the spatial distribution of rocks holds substantial value for studying the geological evolution of landing sites. However, images captured by Mars rovers often exhibit blurred boundaries between rocks and the background, as well as ambiguous textural features of the rocks themselves. These issues complicate the task of distinguishing rocks from the surrounding terrain. Additionally, the scarcity of real Martian rock datasets further exacerbates these challenges, making it increasingly difficult to achieve automatic and accurate rock identification on the Martian surface. To address these challenges and achieve precise rock identification in Mars rover images, this paper proposes a novel model for automatic segmentation of Martian surface rocks based on a convolutional self-attention network. The model implements pixel-level segmentation of images, adopting an encoder-decoder network architecture. The encoder utilizes a Convolutional Neural Network (CNN) to extract image features, while a convolutional self-attention module is incorporated to enhance the model"s ability to understand contextual information within the images and improve its rock detection performance. This module enables the model to focus more effectively on important spatial features across the image, thereby capturing dependencies and contextual relationships between different regions. Finally, the model employs skip connections to facilitate feature fusion, forming a U-shaped decoder network that produces pixel-wise classification outputs, enabling precise segmentation of the images. In this study, we present the Tianwen Mars Surface Image Dataset, a manually annotated dataset of Martian surface imagery captured by the Zhurong rover. We integrate multiple datasets, including the artificially simulated rock datasets Synmars and Simmars6k, as well as the MarsData-v2 dataset from Curiosity rover images, to conduct rigorous testing and validation of the model"s performance. The model"s performance is compared with several other advanced methods, including DeepLabv3+, UNet++, Segformer, and MarsNet, using evaluation metrics such as average pixel accuracy, recall, and Intersection over Union (IoU). The results demonstrate that our proposed model excels in rock extraction, achieving accuracy and recall rates exceeding 90% on the simulated datasets. On real datasets, the model outperforms other methods with the highest accuracy and recall, highlighting its superior performance in rock identification. The experimental comparisons indicate that the model has the potential for accurate and reliable detection of rocks in Mars rover images, which is crucial for both autonomous exploration and scientific research on Mars. The proposed convolutional self-attention module effectively combines the strengths of convolutional operations and Transformer architectures. It enhances the model"s ability to extract contextual information while retaining the capacity to capture detailed features. This dual capability not only improves the model"s segmentation accuracy in areas with dense rock clusters but also enhances its adaptability to regions with blurred boundaries. As a result, the model effectively addresses the challenges associated with accurately identifying surface rocks in the Martian environment, paving the way for more reliable and efficient Mars exploration missions.